Study extra about how we’re making progress in the direction of our sustainability commitments by the Sustainable by design weblog collection, beginning with Sustainable by design: Advancing the sustainability of AI.
Earlier this summer season, my colleague Noelle Walsh printed a weblog detailing how we’re working to preserve water in our datacenter operations: Sustainable by design: Remodeling datacenter water effectivity, as a part of our dedication to our sustainability targets of turning into carbon unfavorable, water optimistic, zero waste, and defending biodiversity.
At Microsoft, we design, construct, and function cloud computing infrastructure spanning the entire stack, from datacenters to servers to customized silicon. This creates distinctive alternatives for orchestrating how the weather work collectively to boost each efficiency and effectivity. We contemplate the work to optimize energy and vitality effectivity a important path to assembly our pledge to be carbon unfavorable by 2030, alongside our work to advance carbon-free electrical energy and carbon elimination.
Discover how we’re advancing the sustainability of AI
Discover our three areas of focus
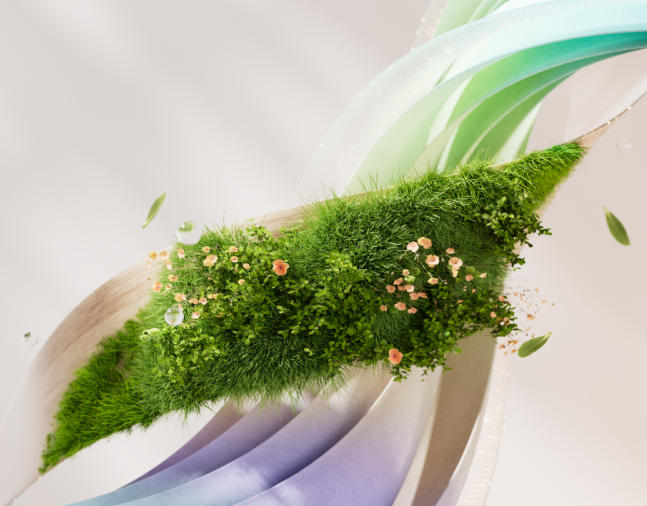
The fast progress in demand for AI innovation to gas the subsequent frontiers of discovery has supplied us with a possibility to revamp our infrastructure methods, from datacenters to servers to silicon, with effectivity and sustainability on the forefront. Along with sourcing carbon-free electrical energy, we’re innovating at each stage of the stack to scale back the vitality depth and energy necessities of cloud and AI workloads. Even earlier than the electrons enter our datacenters, our groups are targeted on how we will maximize the compute energy we will generate from every kilowatt-hour (kWh) of electrical energy.
On this weblog, I’d wish to share some examples of how we’re advancing the ability and vitality effectivity of AI. This features a whole-systems method to effectivity and making use of AI, particularly machine studying, to the administration of cloud and AI workloads.
Driving effectivity from datacenters to servers to silicon
Maximizing {hardware} utilization by sensible workload administration
True to our roots as a software program firm, one of many methods we drive energy effectivity inside our datacenters is thru software program that allows workload scheduling in actual time, so we will maximize the utilization of present {hardware} to fulfill cloud service demand. For instance, we’d see larger demand when individuals are beginning their workday in a single a part of the world, and decrease demand throughout the globe the place others are winding down for the night. In lots of instances, we will align availability for inner useful resource wants, comparable to working AI coaching workloads throughout off-peak hours, utilizing present {hardware} that will in any other case be idle throughout that timeframe. This additionally helps us enhance energy utilization.
We use the ability of software program to drive vitality effectivity at each stage of the infrastructure stack, from datacenters to servers to silicon.
Traditionally throughout the trade, executing AI and cloud computing workloads has relied on assigning central processing models (CPUs), graphics processing models (GPUs), and processing energy to every group or workload, delivering a CPU and GPU utilization price of round 50% to 60%. This leaves some CPUs and GPUs with underutilized capability, potential capability that would ideally be harnessed for different workloads. To deal with the utilization problem and enhance workload administration, we’ve transitioned Microsoft’s AI coaching workloads right into a single pool managed by a machine studying know-how referred to as Challenge Forge.
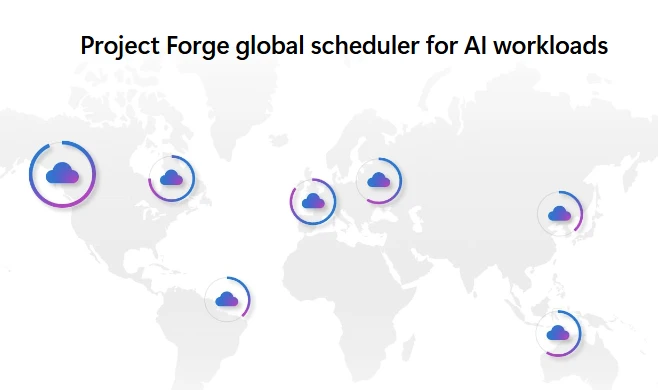
Presently in manufacturing throughout Microsoft providers, this software program makes use of AI to nearly schedule coaching and inferencing workloads, together with clear checkpointing that saves a snapshot of an utility or mannequin’s present state so it may be paused and restarted at any time. Whether or not working on companion silicon or Microsoft’s customized silicon comparable to Maia 100, Challenge Forge has persistently elevated our effectivity throughout Azure to 80 to 90% utilization at scale.
Safely harvesting unused energy throughout our datacenter fleet
One other method we enhance energy effectivity includes putting workloads intelligently throughout a datacenter to soundly harvest any unused energy. Energy harvesting refers to practices that allow us to maximise the usage of our out there energy. For instance, if a workload just isn’t consuming the total quantity of energy allotted to it, that extra energy will be borrowed by and even reassigned to different workloads. Since 2019, this work has recovered roughly 800 megawatts (MW) of electrical energy from present datacenters, sufficient to energy roughly 2.8 million miles pushed by an electrical automotive.1
Over the previous 12 months, at the same time as buyer AI workloads have elevated, our price of enchancment in energy financial savings has doubled. We’re persevering with to implement these finest practices throughout our datacenter fleet as a way to get better and re-allocate unused energy with out impacting efficiency or reliability.
Driving IT {hardware} effectivity by liquid cooling
Along with energy administration of workloads, we’re targeted on decreasing the vitality and water necessities of cooling the chips and the servers that home these chips. With the highly effective processing of contemporary AI workloads comes elevated warmth technology, and utilizing liquid-cooled servers considerably reduces the electrical energy required for thermal administration versus air-cooled servers. The transition to liquid cooling additionally allows us to get extra efficiency out of our silicon, because the chips run extra effectively inside an optimum temperature vary.
A big engineering problem we confronted in rolling out these options was the way to retrofit present datacenters designed for air-cooled servers to accommodate the newest developments in liquid cooling. With customized options such because the “sidekick,” a part that sits adjoining to a rack of servers and circulates fluid like a automotive radiator, we’re bringing liquid cooling options into present datacenters, decreasing the vitality required for cooling whereas growing rack density. This in flip will increase the compute energy we will generate from every sq. foot inside our datacenters.
Study extra and discover sources for cloud and AI effectivity
Keep tuned to be taught extra on this subject, together with how we’re working to deliver promising effectivity analysis out of the lab and into industrial operations. You too can learn extra on how we’re advancing sustainability by our Sustainable by design weblog collection, beginning with Sustainable by design: Advancing the sustainability of AI and Sustainable by design: Remodeling datacenter water effectivity.
For architects, lead builders, and IT choice makers who need to be taught extra about cloud and AI effectivity, we advocate exploring the sustainability steering within the Azure Effectively-Architected Framework. This documentation set aligns to the design ideas of the Inexperienced Software program Basis and is designed to assist clients plan for and meet evolving sustainability necessities and laws across the improvement, deployment, and operations of IT capabilities.
1Equivalency assumptions primarily based on estimates that an electrical automotive can journey on common about 3.5 miles per kilowatt hour (kWh) x 1 hour x 800.