The arrival and progress of generative AI video has prompted many informal observers to predict that machine studying will show the demise of the film business as we all know it – as a substitute, single creators will be capable of create Hollywood-style blockbusters at house, both on native or cloud-based GPU programs.
Is that this attainable? Even whether it is attainable, is it imminent, as so many consider?
That people will finally be capable of create motion pictures, within the type that we all know them, with constant characters, narrative continuity and complete photorealism, is kind of attainable – and maybe even inevitable.
Nonetheless there are a number of actually elementary the reason why this isn’t prone to happen with video programs primarily based on Latent Diffusion Fashions.
This final reality is essential as a result of, in the meanwhile, that class contains each standard text-to-video (T2) and image-to-video (I2V) system out there, together with Minimax, Kling, Sora, Imagen, Luma, Amazon Video Generator, Runway ML, Kaiber (and, so far as we are able to discern, Adobe Firefly’s pending video performance); amongst many others.
Right here, we’re contemplating the prospect of true auteur full-length gen-AI productions, created by people, with constant characters, cinematography, and visible results a minimum of on a par with the present state-of-the-art in Hollywood.
Let’s check out among the largest sensible roadblocks to the challenges concerned.
1: You Can’t Get an Correct Observe-on Shot
Narrative inconsistency is the biggest of those roadblocks. The very fact is that no currently-available video technology system could make a really correct ‘observe on’ shot*.
It is because the denoising diffusion mannequin on the coronary heart of those programs depends on random noise, and this core precept isn’t amenable to reinterpreting precisely the identical content material twice (i.e., from completely different angles, or by creating the earlier shot right into a follow-on shot which maintains consistency with the earlier shot).
The place textual content prompts are used, alone or along with uploaded ‘seed’ photos (multimodal enter), the tokens derived from the immediate will elicit semantically-appropriate content material from the educated latent house of the mannequin.
Nonetheless, additional hindered by the ‘random noise’ issue, it’s going to by no means do it the identical manner twice.
Which means that the identities of individuals within the video will are inclined to shift, and objects and environments won’t match the preliminary shot.
That is why viral clips depicting extraordinary visuals and Hollywood-level output are typically both single photographs, or a ‘showcase montage’ of the system’s capabilities, the place every shot options completely different characters and environments.
Excerpts from a generative AI montage from Marco van Hylckama Vlieg – supply: https://www.linkedin.com/posts/marcovhv_thanks-to-generative-ai-we-are-all-filmmakers-activity-7240024800906076160-nEXZ/
The implication in these collections of advert hoc video generations (which can be disingenuous within the case of business programs) is that the underlying system can create contiguous and constant narratives.
The analogy being exploited here’s a film trailer, which options solely a minute or two of footage from the movie, however offers the viewers motive to consider that all the movie exists.
The one programs which at the moment supply narrative consistency in a diffusion mannequin are those who produce nonetheless photos. These embrace NVIDIA’s ConsiStory, and numerous initiatives within the scientific literature, equivalent to TheaterGen, DreamStory, and StoryDiffusion.
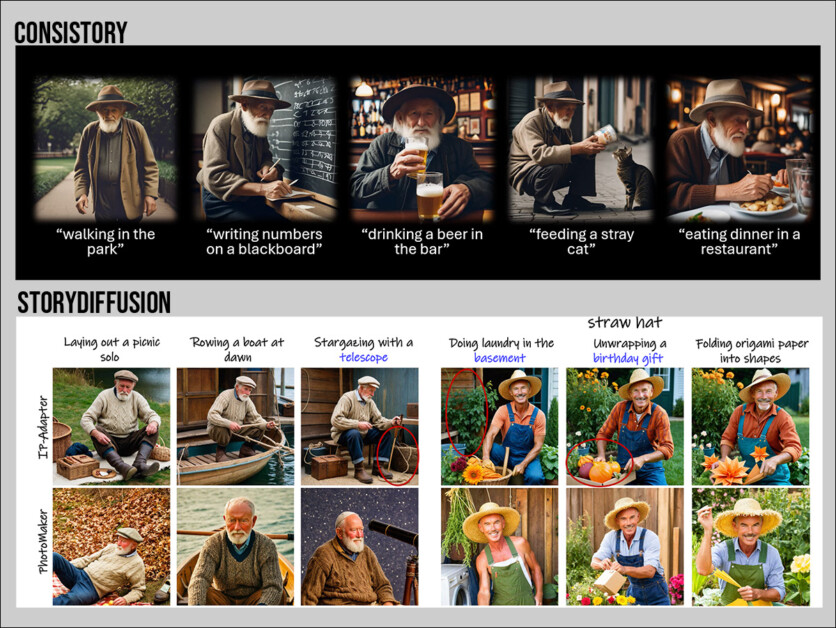
Two examples of ‘static’ narrative continuity, from latest fashions:: Sources: https://analysis.nvidia.com/labs/par/consistory/ and https://arxiv.org/pdf/2405.01434
In idea, one may use a greater model of such programs (not one of the above are actually constant) to create a sequence of image-to-video photographs, which may very well be strung collectively right into a sequence.
On the present state-of-the-art, this strategy doesn’t produce believable follow-on photographs; and, in any case, now we have already departed from the auteur dream by including a layer of complexity.
We will, moreover, use Low Rank Adaptation (LoRA) fashions, particularly educated on characters, issues or environments, to keep up higher consistency throughout photographs.
Nonetheless, if a personality needs to look in a brand new costume, a completely new LoRA will normally should be educated that embodies the character wearing that style (though sub-concepts equivalent to ‘purple gown’ will be educated into particular person LoRAs, along with apposite photos, they aren’t at all times straightforward to work with).
This provides appreciable complexity, even to a gap scene in a film, the place an individual will get away from bed, places on a dressing robe, yawns, appears to be like out the bed room window, and goes to the toilet to brush their enamel.
Such a scene, containing roughly 4-8 photographs, will be filmed in a single morning by typical film-making procedures; on the present state-of-the-art in generative AI, it doubtlessly represents weeks of labor, a number of educated LoRAs (or different adjunct programs), and a substantial quantity of post-processing
Alternatively, video-to-video can be utilized, the place mundane or CGI footage is remodeled by text-prompts into various interpretations. Runway provides such a system, as an illustration.
CGI (left) from Blender, interpreted in a text-aided Runway video-to-video experiment by Mathieu Visnjevec – Supply: https://www.linkedin.com/feed/replace/urn:li:exercise:7240525965309726721/
There are two issues right here: you might be already having to create the core footage, so that you’re already making the film twice, even for those who’re utilizing an artificial system equivalent to UnReal’s MetaHuman.
Should you create CGI fashions (as within the clip above) and use these in a video-to-image transformation, their consistency throughout photographs can’t be relied upon.
It is because video diffusion fashions don’t see the ‘huge image’ – fairly, they create a brand new body primarily based on earlier body/s, and, in some circumstances, think about a close-by future body; however, to match the method to a chess sport, they can not suppose ‘ten strikes forward’, and can’t bear in mind ten strikes behind.
Secondly, a diffusion mannequin will nonetheless battle to keep up a constant look throughout the photographs, even for those who embrace a number of LoRAs for character, surroundings, and lighting fashion, for causes talked about initially of this part.
2: You Cannot Edit a Shot Simply
Should you depict a personality strolling down a road utilizing old-school CGI strategies, and also you determine that you just wish to change some facet of the shot, you’ll be able to regulate the mannequin and render it once more.
If it is a real-life shoot, you simply reset and shoot it once more, with the apposite modifications.
Nonetheless, for those who produce a gen-AI video shot that you just love, however wish to change one facet of it, you’ll be able to solely obtain this by painstaking post-production strategies developed during the last 30-40 years: CGI, rotoscoping, modeling and matting – all labor-intensive and costly, time-consuming procedures.
The best way that diffusion fashions work, merely altering one facet of a text-prompt (even in a multimodal immediate, the place you present an entire supply seed picture) will change a number of elements of the generated output, resulting in a sport of prompting ‘whack-a-mole’.
3: You Can’t Depend on the Legal guidelines of Physics
Conventional CGI strategies supply quite a lot of algorithmic physics-based fashions that may simulate issues equivalent to fluid dynamics, gaseous motion, inverse kinematics (the correct modeling of human motion), fabric dynamics, explosions, and numerous different real-world phenomena.
Nonetheless, diffusion-based strategies, as now we have seen, have quick recollections, and in addition a restricted vary of movement priors (examples of such actions, included within the coaching dataset) to attract on.
In an earlier model of OpenAI’s touchdown web page for the acclaimed Sora generative system, the corporate conceded that Sora has limitations on this regard (although this textual content has since been eliminated):
‘[Sora] could battle to simulate the physics of a posh scene, and should not comprehend particular situations of trigger and impact (for instance: a cookie may not present a mark after a personality bites it).
‘The mannequin may confuse spatial particulars included in a immediate, equivalent to discerning left from proper, or battle with exact descriptions of occasions that unfold over time, like particular digicam trajectories.’
The sensible use of varied API-based generative video programs reveals comparable limitations in depicting correct physics. Nonetheless, sure frequent bodily phenomena, like explosions, seem like higher represented of their coaching datasets.
Some movement prior embeddings, both educated into the generative mannequin or fed in from a supply video, take some time to finish (equivalent to an individual performing a posh and non-repetitive dance sequence in an elaborate costume) and, as soon as once more, the diffusion mannequin’s myopic window of consideration is prone to rework the content material (facial ID, costume particulars, and many others.) by the point the movement has performed out. Nonetheless, LoRAs can mitigate this, to an extent.
Fixing It in Publish
There are different shortcomings to pure ‘single person’ AI video technology, such because the issue they’ve in depicting fast actions, and the overall and way more urgent downside of acquiring temporal consistency in output video.
Moreover, creating particular facial performances is just about a matter of luck in generative video, as is lip-sync for dialogue.
In each circumstances, using ancillary programs equivalent to LivePortrait and AnimateDiff is turning into very fashionable within the VFX group, since this permits the transposition of a minimum of broad facial features and lip-sync to current generated output.
An instance of expression switch (driving video in decrease left) being imposed on a goal video with LivePortrait. The video is from Generative Z TunisiaGenerative. See the full-length model in higher high quality at https://www.linkedin.com/posts/genz-tunisia_digitalcreation-liveportrait-aianimation-activity-7240776811737972736-uxiB/?
Additional, a myriad of advanced options, incorporating instruments such because the Steady Diffusion GUI ComfyUI and the skilled compositing and manipulation software Nuke, in addition to latent house manipulation, permit AI VFX practitioners to achieve larger management over facial features and disposition.
Although he describes the method of facial animation in ComfyUI as ‘torture’, VFX skilled Francisco Contreras has developed such a process, which permits the imposition of lip phonemes and different elements of facial/head depiction”
Steady Diffusion, helped by a Nuke-powered ComfyUI workflow, allowed VFX professional Francisco Contreras to achieve uncommon management over facial elements. For the complete video, at higher decision, go to https://www.linkedin.com/feed/replace/urn:li:exercise:7243056650012495872/
Conclusion
None of that is promising for the prospect of a single person producing coherent and photorealistic blockbuster-style full-length motion pictures, with practical dialogue, lip-sync, performances, environments and continuity.
Moreover, the obstacles described right here, a minimum of in relation to diffusion-based generative video fashions, will not be essentially solvable ‘any minute’ now, regardless of discussion board feedback and media consideration that make this case. The constraints described appear to be intrinsic to the structure.
In AI synthesis analysis, as in all scientific analysis, sensible concepts periodically dazzle us with their potential, just for additional analysis to unearth their elementary limitations.
Within the generative/synthesis house, this has already occurred with Generative Adversarial Networks (GANs) and Neural Radiance Fields (NeRF), each of which finally proved very troublesome to instrumentalize into performant industrial programs, regardless of years of educational analysis in direction of that objective. These applied sciences now present up most ceaselessly as adjunct elements in various architectures.
A lot as film studios could hope that coaching on legitimately-licensed film catalogs may remove VFX artists, AI is definitely including roles to the workforce nowadays.
Whether or not diffusion-based video programs can actually be remodeled into narratively-consistent and photorealistic film mills, or whether or not the entire enterprise is simply one other alchemic pursuit, ought to turn out to be obvious over the following 12 months.
It could be that we’d like a completely new strategy; or it could be that Gaussian Splatting (GSplat), which was developed in the early Nineties and has not too long ago taken off within the picture synthesis house, represents a possible various to diffusion-based video technology.
Since GSplat took 34 years to return to the fore, it is attainable too that older contenders equivalent to NeRF and GANs – and even latent diffusion fashions – are but to have their day.
* Although Kaiber’s AI Storyboard function provides this type of performance, the outcomes I’ve seen are not manufacturing high quality.
Martin Anderson is the previous head of scientific analysis content material at metaphysic.ai
First revealed Monday, September 23, 2024