AI benchmarks have lengthy been the usual for measuring progress in synthetic intelligence. They provide a tangible solution to consider and evaluate system capabilities. However is that this strategy one of the best ways to evaluate AI methods? Andrej Karpathy not too long ago raised considerations in regards to the adequacy of this strategy in a publish on X. AI methods have gotten more and more expert at fixing predefined issues, but their broader utility and flexibility stay unsure. This raises an vital query: Are we holding again AI’s true potential by focusing solely on puzzle-solving benchmarks??
Personally I don’t learn about little benchmarks with puzzles it looks like atari another time. The benchmark I’d search for is nearer to one thing like sum ARR over AI merchandise, unsure if there’s a less complicated / public that captures most of it. I do know the joke is it’s NVDA
— Andrej Karpathy (@karpathy) December 23, 2024
The Drawback with Puzzle-Fixing Benchmarks
LLM benchmarks like MMLU and GLUE have undoubtedly pushed exceptional developments in NLP and Deep Studying. Nonetheless, these benchmarks typically cut back advanced, real-world challenges into well-defined puzzles with clear objectives and analysis standards. Whereas this simplification is sensible for analysis, it could cover deeper capabilities wanted for LLMs to influence society meaningfully.
Karpathy’s publish highlighted a basic difficulty: “Benchmarks have gotten more and more like fixing puzzles.” The responses to his remark reveal widespread settlement throughout the AI group. Many commenters emphasised that the power to generalize and adapt to new, undefined duties is way extra vital than excelling in narrowly outlined benchmarks.
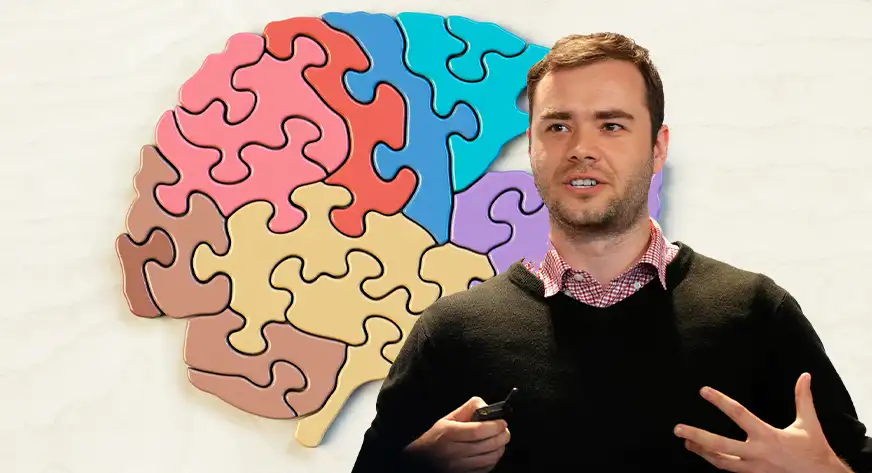
Additionally Learn: Consider a Giant Language Mannequin (LLM)?
Key Challenges with Present Benchmarks
Overfitting to Metrics
AI methods are optimized to carry out effectively on particular datasets or duties, resulting in overfitting. Even when benchmark datasets should not explicitly utilized in coaching, leaks can happen, inflicting the mannequin to inadvertently study benchmark-specific patterns. This hinders its efficiency in broader, real-world purposes.AI methods are optimized to carry out effectively on particular datasets or duties, resulting in overfitting. This doesn’t essentially translate to real-world utility.
Lack of Generalization
Fixing a benchmark activity doesn’t assure that the AI can deal with related, barely completely different issues. For instance, a system educated to caption photographs would possibly wrestle with nuanced descriptions outdoors its coaching information.
Slender Process Definitions
Benchmarks typically concentrate on duties like classification, translation, or summarization. These don’t check broader competencies like reasoning, creativity, or moral decision-making.
Shifting Towards Extra Significant Benchmarks
The constraints of puzzle-solving benchmarks name for a shift in how we consider AI. Beneath are some urged approaches to redefine AI benchmarking:
Actual-World Process Simulation
As an alternative of static datasets, benchmarks might contain dynamic, real-world environments the place AI methods should adapt to altering circumstances. As an example, Google is already engaged on this with initiatives like Genie 2, a large-scale basis world mannequin. Extra particulars will be discovered of their DeepMind weblog and Analytics Vidhya’s article.
- Simulated Brokers: Testing AI in open-ended environments like Minecraft or robotics simulations to guage its problem-solving and flexibility.
- Complicated Eventualities: Deploying AI in real-world industries (e.g., healthcare, local weather modeling) to evaluate its utility in sensible purposes.
Lengthy-Horizon Planning and Reasoning
Benchmarks ought to check AI’s potential to carry out duties requiring long-term planning and reasoning. For instance:
- Multi-step problem-solving that requires an understanding of penalties over time.
- Duties that contain studying new abilities autonomously.
Moral and Social Consciousness
As AI methods more and more work together with people, benchmarks should measure moral reasoning and social understanding. This consists of incorporating security measures and regulatory guardrails to make sure accountable use of AI methods. The latest Purple-teaming Analysis supplies a complete framework for testing AI security and trustworthiness in delicate purposes. Benchmarks should additionally guarantee AI methods make honest, unbiased selections in eventualities involving delicate information and clarify their selections transparently to non-experts. Implementing security measures and regulatory guardrails can mitigate dangers whereas fostering belief in AI purposes. to non-experts.
Generalization Throughout Domains
Benchmarks ought to check an AI’s potential to generalize throughout a number of, unrelated duties. As an example, a single AI system performing effectively in language understanding, picture recognition, and robotics with out specialised fine-tuning for every area.
The Way forward for AI Benchmarks
Because the AI subject evolves, so should its benchmarks. Shifting past puzzle-solving would require collaboration between researchers, practitioners, and policymakers to design benchmarks that align with real-world wants and values. These benchmarks ought to emphasize:
- Adaptability: The power to deal with various, unseen duties.
- Influence: Measuring contributions to significant societal challenges.
- Ethics: Guaranteeing AI aligns with human values and equity.
Finish Observe
Karpathy’s remark challenges us to rethink the aim and design of AI benchmarks. Whereas puzzle-solving benchmarks have pushed unbelievable progress, they might now be holding us again from attaining broader, extra impactful AI methods. The AI group should pivot towards benchmarks that check adaptability, generalization, and real-world utility to unlock AI’s true potential.
The trail ahead won’t be simple, however the reward – AI methods that aren’t solely highly effective but in addition genuinely transformative – is effectively well worth the effort.
What are your ideas on this? Tell us within the remark part under!