NVIDIA’s GeForce RTX 5090 and 5080 GPUs — that are primarily based on the groundbreaking NVIDIA Blackwell structure —supply as much as 8x sooner body charges with NVIDIA DLSS 4 expertise, decrease latency with NVIDIA Reflex 2 and enhanced graphical constancy with NVIDIA RTX neural shaders.
These GPUs had been constructed to speed up the most recent generative AI workloads, delivering as much as 3,352 AI trillion operations per second (TOPS), enabling unimaginable experiences for AI lovers, players, creators and builders.
To assist AI builders and lovers harness these capabilities, NVIDIA on the CES commerce present final month unveiled NVIDIA NIM and AI Blueprints for RTX. NVIDIA NIM microservices are prepackaged generative AI fashions that permit builders and lovers simply get began with generative AI, iterate shortly and harness the ability of RTX for accelerating AI on Home windows PCs. NVIDIA AI Blueprints are reference initiatives that present builders methods to use NIM microservices to construct the following technology of AI experiences.
NIM and AI Blueprints are optimized for GeForce RTX 50 Sequence GPUs. These applied sciences work collectively seamlessly to assist builders and lovers construct, iterate and ship cutting-edge AI experiences on AI PCs.
NVIDIA NIM Accelerates Generative AI on PCs
Whereas AI mannequin growth is quickly advancing, bringing these improvements to PCs stays a problem for many individuals. Fashions posted on platforms like Hugging Face should be curated, tailored and quantized to run on PC. In addition they must be built-in into new AI software programming interfaces (APIs) to make sure compatibility with current instruments, and transformed to optimized inference backends for peak efficiency.
NVIDIA NIM microservices for RTX AI PCs and workstations can ease the complexity of this course of by offering entry to community-driven and NVIDIA-developed AI fashions. These microservices are simple to obtain and connect with through industry-standard APIs and span the important thing modalities important for AI PCs. They’re additionally suitable with a variety of AI instruments and supply versatile deployment choices, whether or not on PCs, in knowledge facilities, or within the cloud.
NIM microservices embrace all the things wanted to run optimized fashions on PCs with RTX GPUs, together with prebuilt engines for particular GPUs, the NVIDIA TensorRT software program growth package (SDK), the open-source NVIDIA TensorRT-LLM library for accelerated inference utilizing Tensor Cores, and extra.
Microsoft and NVIDIA labored collectively to allow NIM microservices and AI Blueprints for RTX in Home windows Subsystem for Linux (WSL2). With WSL2, the identical AI containers that run on knowledge heart GPUs can now run effectively on RTX PCs, making it simpler for builders to construct, take a look at and deploy AI fashions throughout platforms.
As well as, NIM and AI Blueprints harness key improvements of the Blackwell structure that the GeForce RTX 50 collection is constructed on, together with fifth-generation Tensor Cores and help for FP4 precision.
Tensor Cores Drive Subsequent-Gen AI Efficiency
AI calculations are extremely demanding and require huge quantities of processing energy. Whether or not producing photos and movies or understanding language and making real-time choices, AI fashions depend on a whole bunch of trillions of mathematical operations to be accomplished each second. To maintain up, computer systems want specialised {hardware} constructed particularly for AI.
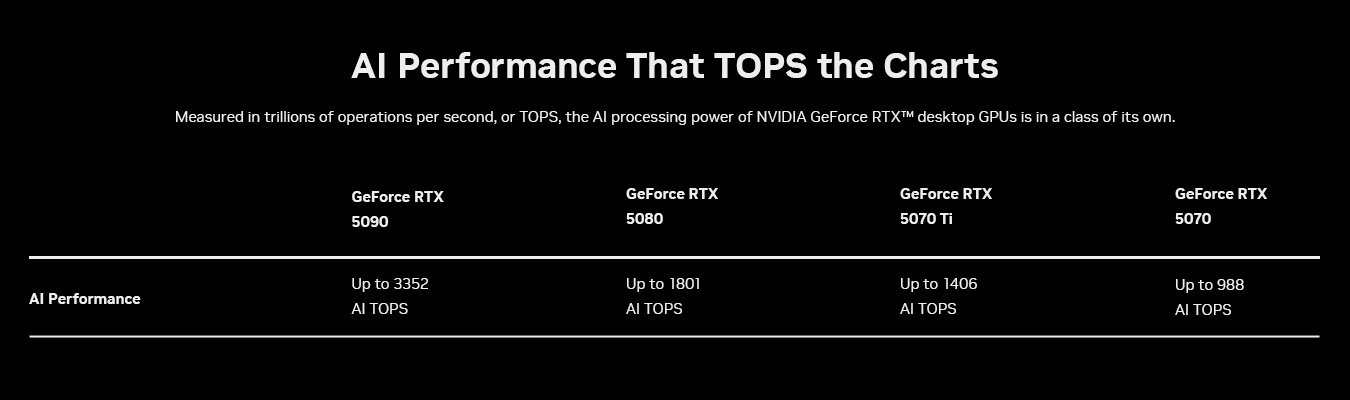
In 2018, NVIDIA GeForce RTX GPUs modified the sport by introducing Tensor Cores — devoted AI processors designed to deal with these intensive workloads. Not like conventional computing cores, Tensor Cores are constructed to speed up AI by performing calculations sooner and extra effectively. This breakthrough helped convey AI-powered gaming, artistic instruments and productiveness purposes into the mainstream.
Blackwell structure takes AI acceleration to the following degree. The fifth-generation Tensor Cores in Blackwell GPUs ship as much as 3,352 AI TOPS to deal with much more demanding AI duties and concurrently run a number of AI fashions. This implies sooner AI-driven experiences, from real-time rendering to clever assistants, that pave the best way for larger innovation in gaming, content material creation and past.
FP4 — Smaller Fashions, Larger Efficiency
One other method to optimize AI efficiency is thru quantization, a method that reduces mannequin sizes, enabling the fashions to run sooner whereas decreasing the reminiscence necessities.
Enter FP4 — a complicated quantization format that enables AI fashions to run sooner and leaner with out compromising output high quality. In contrast with FP16, it reduces mannequin measurement by as much as 60% and greater than doubles efficiency, with minimal degradation.
For instance, Black Forest Labs’ FLUX.1 [dev] mannequin at FP16 requires over 23GB of VRAM, which means it could possibly solely be supported by the GeForce RTX 4090 {and professional} GPUs. With FP4, FLUX.1 [dev] requires lower than 10GB, so it could possibly run domestically on extra GeForce RTX GPUs.
On a GeForce RTX 4090 with FP16, the FLUX.1 [dev] mannequin can generate photos in 15 seconds with simply 30 steps. With a GeForce RTX 5090 with FP4, photos will be generated in simply over 5 seconds.
FP4 is natively supported by the Blackwell structure, making it simpler than ever to deploy high-performance AI on native PCs. It’s additionally built-in into NIM microservices, successfully optimizing fashions that had been beforehand troublesome to quantize. By enabling extra environment friendly AI processing, FP4 helps to convey sooner, smarter AI experiences for content material creation.
AI Blueprints Energy Superior AI Workflows on RTX PCs
NVIDIA AI Blueprints, constructed on NIM microservices, present prepackaged, optimized reference implementations that make it simpler to develop superior AI-powered initiatives — whether or not for digital people, podcast mills or software assistants.
At CES, NVIDIA demonstrated PDF to Podcast, a blueprint that enables customers to transform a PDF right into a enjoyable podcast, and even create a Q&A with the AI podcast host afterwards. This workflow integrates seven completely different AI fashions, all working in sync to ship a dynamic, interactive expertise.
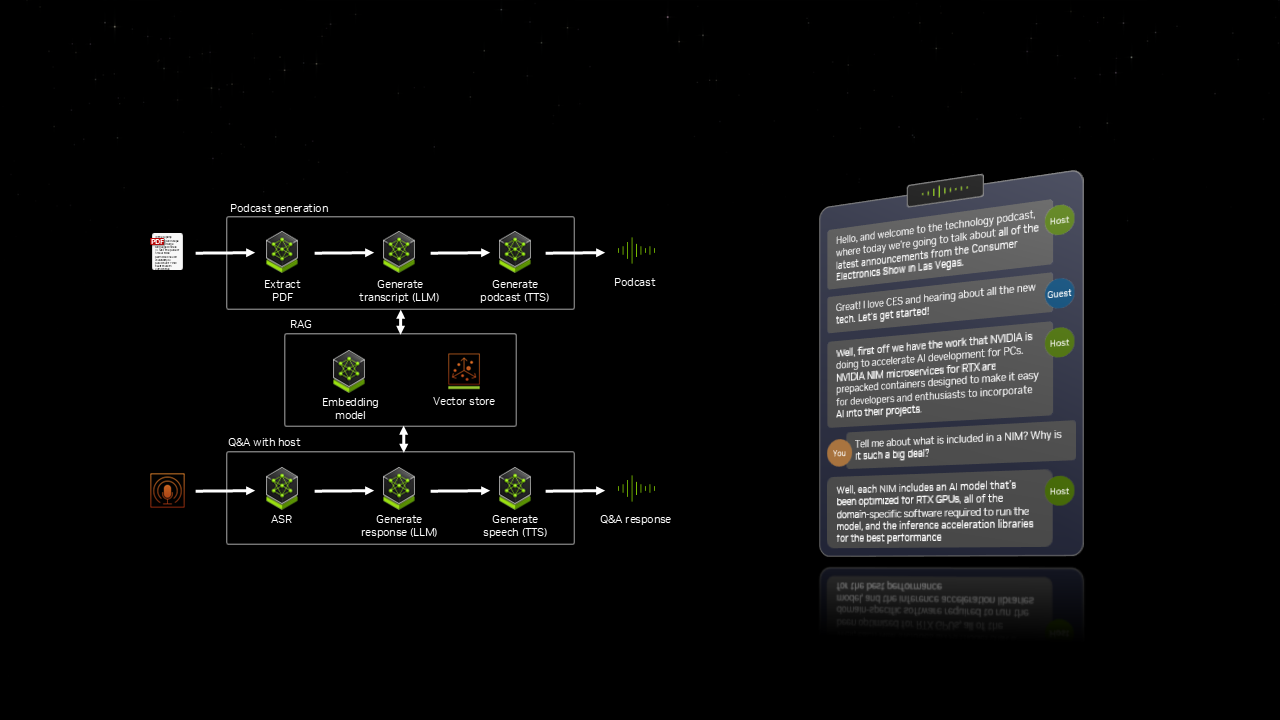
With AI Blueprints, customers can shortly go from experimenting with to growing AI on RTX PCs and workstations.
NIM and AI Blueprints Coming Quickly to RTX PCs and Workstations
Generative AI is pushing the boundaries of what’s doable throughout gaming, content material creation and extra. With NIM microservices and AI Blueprints, the most recent AI developments are not restricted to the cloud — they’re now optimized for RTX PCs. With RTX GPUs, builders and lovers can experiment, construct and deploy AI domestically, proper from their PCs and workstations.
NIM microservices and AI Blueprints are coming quickly, with preliminary {hardware} help for GeForce RTX 50 Sequence, GeForce RTX 4090 and 4080, and NVIDIA RTX 6000 and 5000 skilled GPUs. Further GPUs can be supported sooner or later.