Introduction
Meta has as soon as once more redefined the bounds of synthetic intelligence with the launch of the Section Something Mannequin 2 (SAM-2). This groundbreaking development in laptop imaginative and prescient takes the spectacular capabilities of its predecessor, SAM, to the following degree.
SAM-2 revolutionizes real-time picture and video segmentation, exactly figuring out and segmenting objects. This leap ahead in visible understanding opens up new potentialities for AI functions throughout numerous industries, setting a brand new customary for what’s achievable in laptop imaginative and prescient.
Overview
- Meta’s SAM-2 advances laptop imaginative and prescient with real-time picture and video segmentation, constructing on its predecessor’s capabilities.
- SAM-2 enhances Meta AI’s fashions, extending from static picture segmentation to dynamic video duties with new options and improved efficiency.
- SAM-2 helps video segmentation, unifies structure for picture and video duties, introduces reminiscence elements, and improves effectivity and occlusion dealing with.
- SAM-2 provides real-time video segmentation, zero-shot segmentation for brand spanking new objects, user-guided refinement, occlusion prediction, and a number of masks predictions, excelling in benchmarks.
- SAM-2’s capabilities span video enhancing, augmented actuality, surveillance, sports activities analytics, environmental monitoring, e-commerce, and autonomous autos.
- Regardless of developments, SAM-2 faces challenges in temporal consistency, object disambiguation, high quality element preservation, and long-term reminiscence monitoring, indicating areas for future analysis.
Within the quickly evolving panorama of synthetic intelligence and laptop imaginative and prescient, Meta AI continues to push boundaries with its groundbreaking fashions. Constructing upon the revolutionary Section Something Mannequin (SAM), which we explored in depth in our earlier article “Meta’s Section Something Mannequin: A Leap in Pc Imaginative and prescient,” Meta AI has now launched SAM Meta 2, representing yet one more important leap ahead within the picture and video segmentation know-how.
Our earlier exploration delved into SAM’s revolutionary strategy to picture segmentation, its flexibility in responding to person prompts, and its potential to democratize superior laptop imaginative and prescient throughout numerous industries. SAM’s potential to generalize to new objects and conditions with out extra coaching and the discharge of the intensive Section Something Dataset (SA-1B) set a brand new customary within the area.
Now, with Meta SAM 2, we witness the evolution of this know-how, extending its capabilities from static photos to the dynamic world of video segmentation. This text builds upon our earlier insights, inspecting how Meta SAM 2 not solely enhances the foundational strengths of its predecessor but in addition introduces novel options that promise to reshape our interplay with visible information in movement.
Variations from the Authentic SAM
Whereas SAM 2 builds upon the inspiration laid by its predecessor, it introduces a number of important enhancements:
- Video Functionality: Not like SAM, which was restricted to photographs, SAM 2 can phase objects in movies.
- Unified Structure: SAM 2 makes use of a single mannequin for each picture and video duties, whereas SAM is image-specific.
- Reminiscence Mechanism: The introduction of reminiscence elements permits SAM 2 to trace objects throughout video frames, a function absent within the unique SAM.
- Occlusion Dealing with: SAM 2’s occlusion head permits it to foretell object visibility, a functionality not current in SAM.
- Improved Effectivity: SAM 2 is six occasions sooner than SAM in picture segmentation duties.
- Enhanced Efficiency: SAM 2 outperforms the unique SAM on numerous benchmarks, even in picture segmentation.
SAM-2 Options
Let’s perceive the Options of this mannequin:
- It will probably deal with each picture and video segmentation duties inside a single structure.
- This mannequin can phase objects in movies at roughly 44 frames per second.
- It will probably phase objects it has by no means encountered earlier than, adapt to new visible domains with out extra coaching, or carry out zero-shot segmentation on the brand new photos for objects completely different from its coaching.
- Customers can refine the segmentation on chosen pixels by offering prompts.
- The occlusion head facilitates the mannequin in predicting whether or not an object is seen in a given timeframe.
- SAM-2 outperforms current fashions on numerous benchmarks for each picture and video segmentation duties
What’s New in SAM-2?
Right here’s what SAM-2 has:
- Video Segmentation: an important addition is the power to phase objects in a video, following them throughout all frames and dealing with the occlusion.
- Reminiscence Mechanism: this new model provides a reminiscence encoder, a reminiscence financial institution, and a reminiscence consideration module, which shops and makes use of the knowledge of objects .it additionally helps in person interplay all through the video.
- Streaming Structure: This mannequin processes the video frames one after the other, making it doable to phase lengthy movies in actual time.
- A number of Masks Prediction: SAM 2 can present a number of doable masks when the picture or video is unsure.
- Occlusion Prediction: This new function helps the mannequin to cope with the objects which might be quickly hidden or go away the body.
- Improved Picture Segmentation: SAM 2 is best at segmenting photos than the unique SAM. Whereas it’s superior in video duties.
Demo and Net UI of SAM-2
Meta has additionally launched a web-based demo to indicate SAM 2 capabilities the place customers can
- Add the brief movies or photos
- Section objects in real-time utilizing factors, containers, or masks
- Refine Segmentation throughout video frames
- Apply video results based mostly on the mannequin predictions
- Can add the background impact additionally to a segmented video
Right here’s what the Demo web page appears like, which supplies loads of choices to select from, pin the thing to be traced, and apply completely different results.
The Demo is a good instrument for researchers and builders to discover SAM 2 potential and sensible functions.
Authentic Video
We’re tracing the ball right here.
Segmented video
Analysis on the Mannequin
Analysis and Growth of Meta SAM 2
Mannequin Structure of Meta SAM 2
Meta SAM 2 expands on the unique SAM mannequin, generalizing its potential to deal with photos and movies. The structure is designed to help numerous varieties of prompts (factors, containers, and masks) on particular person video frames, enabling interactive segmentation throughout total video sequences.
Key Parts:
- Picture Encoder: Makes use of a pre-trained Hiera mannequin for environment friendly, real-time processing of video frames.
- Reminiscence Consideration: Situations present body options on previous body data and new prompts utilizing transformer blocks with self-attention and cross-attention mechanisms.
- Immediate Encoder and Masks Decoder: Just like SAM, however tailored for video context. The decoder can predict a number of masks for ambiguous prompts and features a new head to detect object presence in frames.
- Reminiscence Encoder: Generates compact representations of previous predictions and body embeddings.
- Reminiscence Financial institution: This storage space shops data from current frames and prompted frames, together with spatial options and object pointers for semantic data.
Improvements:
- Streaming Strategy: Processes video frames sequentially, permitting for real-time segmentation of arbitrary-length movies.
- Temporal Conditioning: Makes use of reminiscence consideration to include data from previous frames and prompts.
- Flexibility in Prompting: Permits for prompts on any video body, enhancing interactive capabilities.
- Object Presence Detection: Addresses eventualities the place the goal object might not be current in all frames.
Coaching:
The mannequin is skilled on each picture and video information, simulating interactive prompting eventualities. It makes use of sequences of 8 frames, with as much as 2 frames randomly chosen for prompting. This strategy helps the mannequin study to deal with numerous prompting conditions and propagate segmentation throughout video frames successfully.
This structure permits Meta SAM 2 to supply a extra versatile and interactive expertise for video segmentation duties. It builds upon the strengths of the unique SAM mannequin whereas addressing the distinctive challenges of video information.
Promptable Visible Segmentation: Increasing SAM’s Capabilities to Video
Promptable Visible Segmentation (PVS) represents a major evolution of the Section Something (SA) job, extending its capabilities from static photos to the dynamic realm of video. This development permits for interactive segmentation throughout total video sequences, sustaining the pliability and responsiveness that made SAM revolutionary.
Within the PVS framework, customers can work together with any video body utilizing numerous immediate sorts, together with clicks, containers, or masks. The mannequin then segments and tracks the required object all through the whole video. This interplay maintains the instantaneous response on the prompted body, just like SAM’s efficiency on static photos, whereas additionally producing segmentations for the whole video in close to real-time.
Key options of PVS embrace:
- Multi-frame Interplay: PVS permits prompts on any body, not like conventional video object segmentation duties that usually depend on first-frame annotations.
- Numerous Immediate Varieties: Customers can make use of clicks, masks, or bounding containers as prompts, enhancing flexibility.
- Actual-time Efficiency: The mannequin offers prompt suggestions on the prompted body and swift segmentation throughout the whole video.
- Give attention to Outlined Objects: Just like SAM, PVS targets objects with clear visible boundaries, excluding ambiguous areas.
PVS bridges a number of associated duties in each picture and video domains:
- It encompasses the Section Something job for static photos as a particular case.
- It extends past conventional semi-supervised and interactive video object segmentation duties, usually restricted to particular prompts or first-frame annotations.
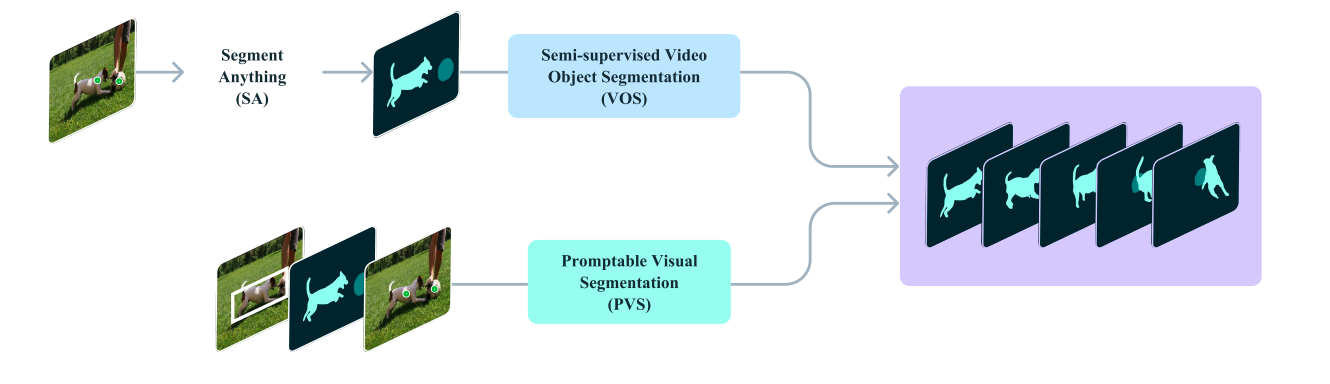
The evolution of Meta SAM 2 concerned a three-phase analysis course of, every part bringing important enhancements in annotation effectivity and mannequin capabilities:
1st Section: Foundational Annotation with SAM
- Strategy: Used image-based interactive SAM for frame-by-frame annotation
- Course of: Annotators manually segmented objects at 6 FPS utilizing SAM and enhancing instruments
- Outcomes:
- 16,000 masklets collected throughout 1,400 movies
- Common annotation time: 37.8 seconds per body
- Produced high-quality spatial annotations however was time-intensive
2nd Section: Introducing SAM 2 Masks
- Enchancment: Built-in SAM 2 Masks for temporal masks propagation
- Course of:
- Preliminary body annotated with SAM
- SAM 2 Masks propagated annotations to subsequent frames
- Annotators refined predictions as wanted
- Outcomes:
- 63,500 masklets collected
- Annotation time lowered to 7.4 seconds per body (5.1x speed-up)
- The mannequin was retrained twice throughout this part
third Section: Full Implementation of SAM 2
- Options: Unified mannequin for interactive picture segmentation and masks propagation
- Developments:
- Accepts numerous immediate sorts (factors, masks)
- Makes use of temporal reminiscence for improved predictions
- Outcomes:
- 197,000 masklets collected
- Annotation time was additional lowered to 4.5 seconds per body (8.4x speed-up from Section 1)
- The mannequin was retrained 5 occasions with newly collected information
Right here’s a comparability between phases :
Key Enhancements:
- Effectivity: Annotation time decreased from 37.8 to 4.5 seconds per body throughout phases.
- Versatility: Developed from frame-by-frame annotation to seamless video segmentation.
- Interactivity: Progressed to a system requiring solely occasional refinement clicks.
- Mannequin Enhancement: Steady retraining with new information improved efficiency.
This phased strategy showcases the iterative growth of Meta SAM 2, highlighting important developments in each the mannequin’s capabilities and the effectivity of the annotation course of. The analysis demonstrates a transparent development in direction of a extra sturdy, versatile, and user-friendly video segmentation instrument.
The analysis paper demonstrates a number of important developments achieved by Meta SAM 2:
- Meta SAM 2 outperforms current approaches throughout 17 zero-shot video datasets, requiring roughly 66% fewer human-in-the-loop interactions for interactive video segmentation.
- It surpasses the unique SAM on its 23-dataset zero-shot benchmark suite whereas working six occasions sooner for picture segmentation duties.
- Meta SAM 2 excels on established video object segmentation benchmarks like DAVIS, MOSE, LVOS, and YouTube-VOS, setting new state-of-the-art requirements.
- The mannequin achieves an inference pace of roughly 44 frames per second, offering a real-time person expertise. When used for video segmentation annotation, Meta SAM 2 is 8.4 occasions sooner than handbook per-frame annotation with the unique SAM.
- To make sure equitable efficiency throughout numerous person teams, the researchers carried out equity evaluations of Meta SAM 2:
The mannequin exhibits minimal efficiency discrepancy in video segmentation throughout perceived gender teams.
These outcomes underscore Meta SAM 2’s pace, accuracy, and flexibility developments throughout numerous segmentation duties whereas demonstrating its constant efficiency throughout completely different demographic teams. This mixture of technical prowess and equity concerns positions Meta SAM 2 as a major step ahead in visible segmentation.
The Section Something 2 mannequin is constructed upon a sturdy and numerous dataset known as SA-V (Section Something – Video). This dataset represents a major development in laptop imaginative and prescient, notably for coaching general-purpose object segmentation fashions from open-world movies.
SA-V contains an intensive assortment of 51,000 numerous movies and 643,000 spatio-temporal segmentation masks known as masklets. This huge-scale dataset is designed to cater to a variety of laptop imaginative and prescient analysis functions working below the permissive CC BY 4.0 license.
Key traits of the SA-V dataset embrace:
- Scale and Variety: With 51,000 movies and a median of 12.61 masklets per video, SA-V provides a wealthy and various information supply. The movies cowl numerous topics, from areas and objects to complicated scenes, making certain complete protection of real-world eventualities.
- Excessive-High quality Annotations: The dataset includes a mixture of human-generated and AI-assisted annotations. Out of the 643,000 masklets, 191,000 had been created by means of SAM 2-assisted handbook annotation, whereas 452,000 had been robotically generated by SAM 2 and verified by human annotators.
- Class-Agnostic Strategy: SA-V adopts a class-agnostic annotation technique, specializing in masks annotations with out particular class labels. This strategy enhances the mannequin’s versatility in segmenting numerous objects and scenes.
- Excessive-Decision Content material: The common video decision within the dataset is 1401×1037 pixels, offering detailed visible data for efficient mannequin coaching.
- Rigorous Validation: All 643,000 masklet annotations underwent evaluation and validation by human annotators, making certain excessive information high quality and reliability.
- Versatile Format: The dataset offers masks in numerous codecs to go well with numerous wants – COCO run-length encoding (RLE) for the coaching set and PNG format for validation and check units.
The creation of SA-V concerned a meticulous information assortment, annotation, and validation course of. Movies had been sourced by means of a contracted third-party firm and thoroughly chosen based mostly on content material relevance. The annotation course of leveraged each the capabilities of the SAM 2 mannequin and the experience of human annotators, leading to a dataset that balances effectivity with accuracy.
Listed below are instance movies from the SA-V dataset with masklets overlaid (each handbook and automated). Every masklet is represented by a singular colour, and every row shows frames from a single video, with a 1-second interval between frames:
You possibly can obtain the SA-V dataset instantly from Meta AI. The dataset is accessible on the following hyperlink:
To entry the dataset, you should present sure data in the course of the obtain course of. This usually contains particulars about your supposed use of the dataset and settlement to the phrases of use. When downloading and utilizing the dataset, it’s essential to rigorously learn and adjust to the licensing phrases (CC BY 4.0) and utilization tips supplied by Meta AI.
Whereas Meta SAM 2 represents a major development in video segmentation know-how, it’s essential to acknowledge its present limitations and areas for future enchancment:
1. Temporal Consistency
The mannequin might wrestle to keep up constant object monitoring in eventualities involving speedy scene adjustments or prolonged video sequences. As an example, Meta SAM 2 would possibly lose observe of a selected participant throughout a fast-paced sports activities occasion with frequent digital camera angle shifts.
2. Object Disambiguation
The mannequin can sometimes misidentify the goal in complicated environments with a number of related objects. For instance, a busy city road scene would possibly confuse completely different vehicles of the identical mannequin and colour.
3. Tremendous Element Preservation
Meta SAM 2 might not all the time seize intricate particulars precisely for objects in swift movement. This might be noticeable when making an attempt to phase the person feathers of a hen in flight.
4. Multi-Object Effectivity
Whereas able to segmenting a number of objects concurrently, the mannequin’s efficiency decreases because the variety of tracked objects will increase. This limitation turns into obvious in eventualities like crowd evaluation or multi-character animation.
5. Lengthy-term Reminiscence
The mannequin’s potential to recollect and observe objects over prolonged intervals in longer movies is proscribed. This might pose challenges in functions like surveillance or long-form video enhancing.
6. Generalization to Unseen Objects
Meta SAM 2 might wrestle with extremely uncommon or novel objects that considerably differ from its coaching information regardless of its broad coaching.
7. Interactive Refinement Dependency
In difficult instances, the mannequin typically depends on extra person prompts for correct segmentation, which might not be superb for totally automated functions.
8. Computational Assets
Whereas sooner than its predecessor, Meta SAM 2 nonetheless requires substantial computational energy for real-time efficiency, probably limiting its use in resource-constrained environments.
Future analysis instructions may improve temporal consistency, enhance high quality element preservation in dynamic scenes, and develop extra environment friendly multi-object monitoring mechanisms. Moreover, exploring methods to scale back the necessity for handbook intervention and increasing the mannequin’s potential to generalize to a wider vary of objects and eventualities can be precious. As the sector progresses, addressing these limitations can be essential in realizing the total potential of AI-driven video segmentation know-how.
The event of Meta SAM 2 opens up thrilling potentialities for the way forward for AI and laptop imaginative and prescient:
- Enhanced AI-Human Collaboration: As fashions like Meta SAM 2 change into extra subtle, we will anticipate to see extra seamless collaboration between AI programs and human customers in visible evaluation duties.
- Developments in Autonomous Techniques: The improved real-time segmentation capabilities may considerably improve the notion programs of autonomous autos and robots, permitting for extra correct and environment friendly navigation and interplay with their environments.
- Evolution of Content material Creation: The know-how behind Meta SAM 2 may result in extra superior instruments for video enhancing and content material creation, probably remodeling industries like movie, tv, and social media.
- Progress in Medical Imaging: Future iterations of this know-how may revolutionize medical picture evaluation, enabling extra correct and sooner analysis throughout numerous medical fields.
- Moral AI Growth: The equity evaluations carried out on Meta SAM 2 set a precedent for contemplating demographic fairness in AI mannequin growth, probably influencing future AI analysis and growth practices.
Meta SAM 2’s capabilities open up a variety of potential functions throughout numerous industries:
- Video Enhancing and Put up-Manufacturing: The mannequin’s potential to effectively phase objects in video may streamline enhancing processes, making complicated duties like object removing or substitute extra accessible.
- Augmented Actuality: Meta SAM 2’s real-time segmentation capabilities may improve AR functions, permitting for extra correct and responsive object interactions in augmented environments.
- Surveillance and Safety: The mannequin’s potential to trace and phase objects throughout video frames may enhance safety programs, enabling extra subtle monitoring and risk detection.
- Sports activities Analytics: In sports activities broadcasting and evaluation, Meta SAM 2 may observe participant actions, analyze sport methods, and create extra partaking visible content material for viewers.
- Environmental Monitoring: The mannequin might be employed to trace and analyze adjustments in landscapes, vegetation, or wildlife populations over time for ecological research or city planning.
- E-commerce and Digital Strive-Ons: The know-how may improve digital try-on experiences in on-line buying, permitting for extra correct and life like product visualizations.
- Autonomous Automobiles: Meta SAM 2’s segmentation capabilities may enhance object detection and scene understanding in self-driving automotive programs, probably enhancing security and navigation.
These functions showcase the flexibility of Meta SAM 2 and spotlight its potential to drive innovation throughout a number of sectors, from leisure and commerce to scientific analysis and public security.
Conclusion
Meta SAM 2 represents a major leap ahead in visible segmentation, constructing upon the inspiration laid by its predecessor. This superior mannequin demonstrates outstanding versatility, dealing with each picture and video segmentation duties with elevated effectivity and accuracy. Its potential to course of video frames in actual time whereas sustaining high-quality segmentation marks a brand new milestone in laptop imaginative and prescient know-how.
The mannequin’s improved efficiency throughout numerous benchmarks, coupled with its lowered want for human intervention, showcases the potential of AI to revolutionize how we work together with and analyze visible information. Whereas Meta SAM 2 isn’t with out its limitations, similar to challenges with speedy scene adjustments and high quality element preservation in dynamic eventualities, it units a brand new customary for promptable visible segmentation. It paves the way in which for future developments within the area.
Regularly Requested Questions
Ans. Meta SAM 2 is a sophisticated AI mannequin for picture and video segmentation. Not like the unique SAM, which was restricted to photographs, SAM 2 can phase objects in each photos and movies. It’s six occasions sooner than SAM for picture segmentation, can course of movies at about 44 frames per second, and contains new options like a reminiscence mechanism and occlusion prediction.
Ans. SAM 2’s key options embrace:
– Unified structure for each picture and video segmentation
– Actual-time video segmentation capabilities
– Zero-shot segmentation for brand spanking new objects
– Consumer-guided refinement of segmentation
– Occlusion prediction
– A number of masks prediction for unsure instances
– Improved efficiency on numerous benchmarks
Ans. SAM 2 makes use of a streaming structure to course of video frames sequentially in actual time. It incorporates a reminiscence mechanism (together with a reminiscence encoder, reminiscence financial institution, and reminiscence consideration module) to trace objects throughout frames and deal with occlusions. This permits it to phase and observe objects all through a video, even when quickly hidden or leaving the body.
Ans. SAM 2 was skilled on the SA-V (Section Something – Video) dataset. This dataset contains 51,000 numerous movies with 643,000 spatio-temporal segmentation masks (known as masklets). The dataset combines human-generated and AI-assisted annotations, all validated by human annotators, and is accessible below a CC BY 4.0 license.