Whereas everybody’s been buzzing about AI brokers and automation, AMD and Johns Hopkins College have been engaged on enhancing how people and AI collaborate in analysis. Their new open-source framework, Agent Laboratory, is a whole reimagining of how scientific analysis may be accelerated by means of human-AI teamwork.
After taking a look at quite a few AI analysis frameworks, Agent Laboratory stands out for its sensible strategy. As a substitute of making an attempt to exchange human researchers (like many current options), it focuses on supercharging their capabilities by dealing with the time-consuming facets of analysis whereas maintaining people within the driver’s seat.
The core innovation right here is straightforward however highly effective: Somewhat than pursuing absolutely autonomous analysis (which frequently results in questionable outcomes), Agent Laboratory creates a digital lab the place a number of specialised AI brokers work collectively, every dealing with completely different facets of the analysis course of whereas staying anchored to human steering.
Breaking Down the Digital Lab
Consider Agent Laboratory as a well-orchestrated analysis workforce, however with AI brokers enjoying specialised roles. Identical to an actual analysis lab, every agent has particular tasks and experience:
- A PhD agent tackles literature critiques and analysis planning
- Postdoc brokers assist refine experimental approaches
- ML Engineer brokers deal with the technical implementation
- Professor brokers consider and rating analysis outputs
What makes this method significantly attention-grabbing is its workflow. Not like conventional AI instruments that function in isolation, Agent Laboratory creates a collaborative surroundings the place these brokers work together and construct upon one another’s work.
The method follows a pure analysis development:
- Literature Evaluate: The PhD agent scours educational papers utilizing the arXiv API, gathering and organizing related analysis
- Plan Formulation: PhD and postdoc brokers workforce as much as create detailed analysis plans
- Implementation: ML Engineer brokers write and check code
- Evaluation & Documentation: The workforce works collectively to interpret outcomes and generate complete reviews
However this is the place it will get actually sensible: The framework is compute-flexible, that means researchers can allocate sources primarily based on their entry to computing energy and funds constraints. This makes it a device designed for real-world analysis environments.
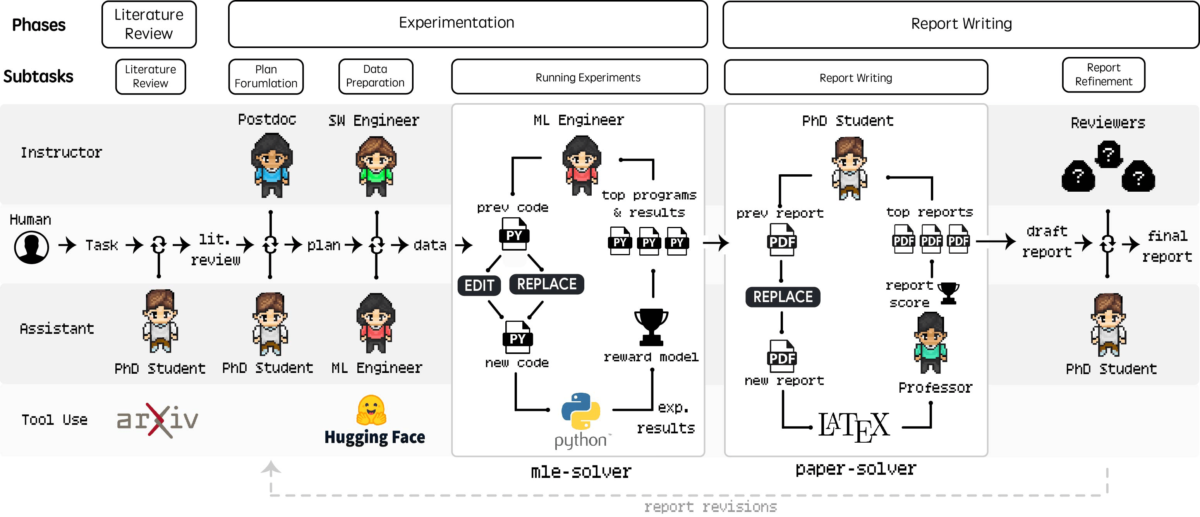
Schmidgall et al.
The Human Issue: The place AI Meets Experience
Whereas Agent Laboratory packs spectacular automation capabilities, the actual magic occurs in what they name “co-pilot mode.” On this setup, researchers can present suggestions at every stage of the method, creating a real collaboration between human experience and AI help.
The co-pilot suggestions knowledge reveals some compelling insights. Within the autonomous mode, Agent Laboratory-generated papers scored a mean of three.8/10 in human evaluations. However when researchers engaged in co-pilot mode, these scores jumped to 4.38/10. What is especially attention-grabbing is the place these enhancements confirmed up – papers scored considerably larger in readability (+0.23) and presentation (+0.33).
However right here is the truth verify: even with human involvement, these papers nonetheless scored about 1.45 factors beneath the common accepted NeurIPS paper (which sits at 5.85). This isn’t a failure, however it’s a essential studying about how AI and human experience want to enrich one another.
The analysis revealed one thing else fascinating: AI reviewers persistently rated papers about 2.3 factors larger than human reviewers. This hole highlights why human oversight stays essential in analysis analysis.
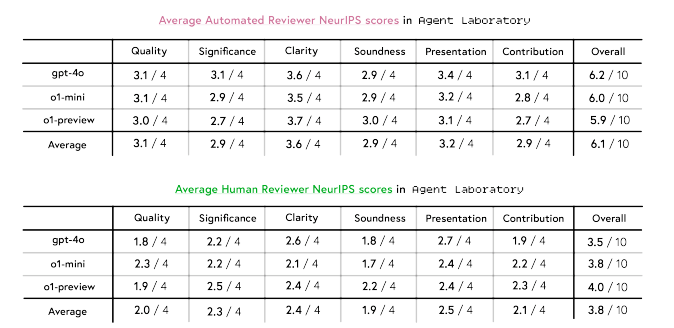
Schmidgall et al.
Breaking Down the Numbers
What actually issues in a analysis surroundings? The associated fee and efficiency. Agent Laboratory’s strategy to mannequin comparability reveals some shocking effectivity positive aspects on this regard.
GPT-4o emerged because the pace champion, finishing the complete workflow in simply 1,165.4 seconds – that is 3.2x quicker than o1-mini and 5.3x quicker than o1-preview. However what’s much more necessary is that it solely prices $2.33 per paper. In comparison with earlier autonomous analysis strategies that value round $15, we’re taking a look at an 84% value discount.
Taking a look at mannequin efficiency:
- o1-preview scored highest in usefulness and readability
- o1-mini achieved the very best experimental high quality scores
- GPT-4o lagged in metrics however led in cost-efficiency
The actual-world implications listed here are important.
Researchers can now select their strategy primarily based on their particular wants:
- Want speedy prototyping? GPT-4o gives pace and value effectivity
- Prioritizing experimental high quality? o1-mini may be your finest wager
- Searching for probably the most polished output? o1-preview exhibits promise
This flexibility means analysis groups can adapt the framework to their sources and necessities, somewhat than being locked right into a one-size-fits-all resolution.
A New Chapter in Analysis
After wanting into Agent Laboratory’s capabilities and outcomes, I’m satisfied that we’re taking a look at a big shift in how analysis will probably be performed. However it isn’t the narrative of substitute that usually dominates headlines – it’s one thing way more nuanced and highly effective.
Whereas Agent Laboratory’s papers aren’t but hitting high convention requirements on their very own, they’re creating a brand new paradigm for analysis acceleration. Consider it like having a workforce of AI analysis assistants who by no means sleep, every specializing in several facets of the scientific course of.
The implications for researchers are profound:
- Time spent on literature critiques and primary coding might be redirected to inventive ideation
- Analysis concepts which may have been shelved on account of useful resource constraints turn into viable
- The power to quickly prototype and check hypotheses might result in quicker breakthroughs
Present limitations, just like the hole between AI and human evaluate scores, are alternatives. Every iteration of those programs brings us nearer to extra refined analysis collaboration between people and AI.
Wanting forward, I see three key developments that might reshape scientific discovery:
- Extra refined human-AI collaboration patterns will emerge as researchers be taught to leverage these instruments successfully
- The associated fee and time financial savings might democratize analysis, permitting smaller labs and establishments to pursue extra bold initiatives
- The speedy prototyping capabilities might result in extra experimental approaches in analysis
The important thing to maximizing this potential? Understanding that Agent Laboratory and comparable frameworks are instruments for amplification, not automation. The way forward for analysis is not about selecting between human experience and AI capabilities – it is about discovering revolutionary methods to mix them.