China is advancing quickly in generative AI, constructing on successes like DeepSeek fashions and Kimi k1.5 in language fashions. Now, it’s main the imaginative and prescient area with OmniHuman and Goku excelling in 3D modeling and video synthesis. With Step-Video-T2V, China immediately challenges prime text-to-video fashions like Sora, Veo 2, and Film Gen. Developed by Stepfun AI, Step-Video-T2V is a 30B-parameter mannequin that generates high-quality, 204-frame movies. It leverages a Video-VAE, bilingual encoders, and a 3D-attention DiT to set a brand new video era commonplace. Does it handle text-to-video’s core challenges? Let’s dive in.
Challenges in Textual content-to-Video Fashions
Whereas text-to-video fashions have come a good distance, they nonetheless face elementary hurdles:
- Complicated Motion Sequences – Present fashions battle to generate reasonable movies that comply with intricate motion sequences, comparable to a gymnast performing flips or a basketball bouncing realistically.
- Physics and Causality – Most diffusion-based fashions fail to simulate the actual world successfully. Object interactions, gravity, and bodily legal guidelines are sometimes neglected.
- Instruction Following – Fashions regularly miss key particulars in consumer prompts, particularly when coping with uncommon ideas (e.g., a penguin and an elephant in the identical video).
- Computational Prices – Producing high-resolution, long-duration movies is extraordinarily resource-intensive, limiting accessibility for researchers and creators.
- Captioning and Alignment – Video fashions depend on huge datasets, however poor video captioning ends in weak immediate adherence, resulting in hallucinated content material.
How Step-Video-T2V is Fixing These Issues?
Step-Video-T2V tackles these challenges with a number of improvements:
- Deep Compression Video-VAE: Achieves 16×16 spatial and 8x temporal compression, considerably lowering computational necessities whereas sustaining excessive video high quality.
- Bilingual Textual content Encoders: Integrates Hunyuan-CLIP and Step-LLM, permitting the mannequin to course of prompts successfully in each Chinese language and English.
- 3D Full-Consideration DiT: As an alternative of conventional spatial-temporal consideration, this strategy enhances movement continuity and scene consistency.
- Video-DPO (Direct Desire Optimization): Incorporates human suggestions loops to cut back artifacts, enhance realism, and align generated content material with consumer expectations.
Mannequin Structure
The Step-Video-T2V mannequin structure is structured round a three-part pipeline to successfully course of textual content prompts and generate high-quality movies. The mannequin integrates a bilingual textual content encoder, a Variational Autoencoder (Video-VAE), and a Diffusion Transformer (DiT) with 3D Consideration, setting it aside from conventional text-to-video fashions.
1. Textual content Encoding with Bilingual Understanding
On the enter stage, Step-Video-T2V employs two highly effective bilingual textual content encoders:
- Hunyuan-CLIP: A vision-language mannequin optimized for semantic alignment between textual content and pictures.
- Step-LLM: A big language mannequin specialised in understanding advanced directions in each Chinese language and English.
These encoders course of the consumer immediate and convert it right into a significant latent illustration, guaranteeing that the mannequin precisely follows directions.
2. Variational Autoencoder (Video-VAE) for Compression
Producing lengthy, high-resolution movies is computationally costly. Step-Video-T2V tackles this problem with a deep compression Variational Autoencoder (Video-VAE) that reduces video knowledge effectively:
- Spatial compression (16×16) and temporal compression (8x) cut back video measurement whereas preserving movement particulars.
- This allows longer sequences (204 frames) with decrease compute prices than earlier fashions.
3. Diffusion Transformer (DiT) with 3D Full Consideration
The core of Step-Video-T2V is its Diffusion Transformer (DiT) with 3D Full Consideration, which considerably improves movement smoothness and scene coherence.
The ith block of the DiT consists of a number of parts that refine the video era course of:
Key Elements of Every Transformer Block
- Cross-Consideration: Ensures higher text-to-video alignment by conditioning the generated frames on the textual content embedding.
- Self-Consideration (with RoPE-3D): Makes use of Rotary Positional Encoding (RoPE-3D) to reinforce spatial-temporal understanding, guaranteeing that objects transfer naturally throughout frames.
- QK-Norm (Question-Key Normalization): Improves the soundness of consideration mechanisms, lowering inconsistencies in object positioning.
- Gate Mechanisms: These adaptive gates regulate data circulation, stopping overfitting to particular patterns and bettering generalization.
- Scale/Shift Operations: Normalize and fine-tune intermediate representations, guaranteeing easy transitions between video frames.
4. Adaptive Layer Normalization (AdaLN-Single)
- The mannequin additionally contains Adaptive Layer Normalization (AdaLN-Single), which adjusts activations dynamically primarily based on the timestep (t).
- This ensures temporal consistency throughout the video sequence.
How Does Step-Video-T2V Work?
The Step-Video-T2V mannequin is a cutting-edge text-to-video AI system that generates high-quality motion-rich movies primarily based on textual descriptions. The working mechanism includes a number of subtle AI methods to make sure easy movement, adherence to prompts, and reasonable output. Let’s break it down step-by-step:
1. Person Enter (Textual content Encoding)
- The mannequin begins by processing consumer enter, which is a textual content immediate describing the specified video.
- That is accomplished utilizing bilingual textual content encoders (e.g., Hunyuan-CLIP and Step-LLM).
- The bilingual functionality ensures that prompts in each English and Chinese language will be understood precisely.
2. Latent Illustration (Compression with Video-VAE)
- Video era is computationally heavy, so the mannequin employs a Variational Autoencoder (VAE) specialised for video compression, referred to as Video-VAE.
- Perform of Video-VAE:
- Compresses video frames right into a lower-dimensional latent house, considerably lowering computational prices.
- Maintains key video high quality features, comparable to movement continuity, textures, and object particulars.
- Makes use of a 16×16 spatial and 8x temporal compression, making the mannequin environment friendly whereas preserving excessive constancy.
3. Denoising Course of (Diffusion Transformer with 3D Full Consideration)
- After acquiring the latent illustration, the subsequent step is the denoising course of, which refines the video frames.
- That is accomplished utilizing a Diffusion Transformer (DiT), a sophisticated mannequin designed for producing extremely reasonable movies.
- Key innovation:
- The Diffusion Transformer applies 3D Full Consideration, a robust mechanism that focuses on spatial, temporal, and movement dynamics.
- Using Circulate Matching helps improve the motion consistency throughout frames, guaranteeing smoother video transitions.
4. Optimization (Superb-Tuning and Video-DPO Coaching)
The generated video undergoes an optimization part, making it extra correct, coherent, and visually interesting. This includes:
- Superb-tuning the mannequin with high-quality knowledge to enhance its potential to comply with advanced prompts.
- Video-DPO (Direct Desire Optimization) coaching, which contains human suggestions to:
- Scale back undesirable artifacts.
- Enhance realism in movement and textures.
- Align video era with consumer expectations.
5. Ultimate Output (Excessive-High quality 204-Body Video)
- The ultimate video is 204 frames lengthy, which means it gives a vital length for storytelling.
- Excessive-resolution era ensures crisp visuals and clear object rendering.
- Sturdy movement realism means the video maintains easy and pure motion, making it appropriate for advanced scenes like human gestures, object interactions, and dynamic backgrounds.
Benchmarking Towards Opponents
Step-Video-T2V is evaluated on Step-Video-T2V-Eval, a 128-prompt benchmark overlaying sports activities, meals, surroundings, surrealism, folks, and animation. In contrast towards main fashions, it delivers state-of-the-art efficiency in movement dynamics and realism.
- Outperforms HunyuanVideo in general video high quality and smoothness.
- Rivals Film Gen Video however lags in fine-grained aesthetics attributable to restricted high-quality labeled knowledge.
- Beats Runway Gen-3 Alpha in movement consistency however barely lags in cinematic enchantment.
- Challenges High Chinese language business fashions (T2VTopA and T2VTopB) however falls brief in aesthetic high quality attributable to decrease decision (540P vs. 1080P).
Efficiency Metrics
Step-Video-T2V introduces new analysis standards:
- Instruction Following – Measures how effectively the generated video aligns with the immediate.
- Movement Smoothness – Charges the pure circulation of actions within the video.
- Bodily Plausibility – Evaluates whether or not actions comply with the legal guidelines of physics.
- Aesthetic Attraction – Judges the inventive and visible high quality of the video.
In human evaluations, Step-Video-T2V constantly outperforms rivals in movement smoothness and bodily plausibility, making it one of the crucial superior open-source fashions.
Learn how to Entry Step-Video-T2V?
Step 1: Go to the official web site right here.
Step 2: Join utilizing your cell quantity.
Be aware: Presently, registrations are open just for a restricted variety of international locations. Sadly, it isn’t accessible in India, so I couldn’t join. Nonetheless, you’ll be able to attempt when you’re situated in a supported area.
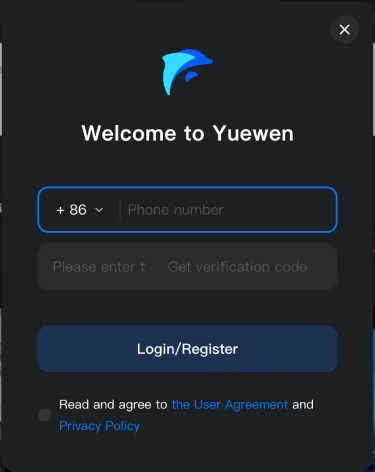
Step 3: Add in your immediate and begin producing superb movies!
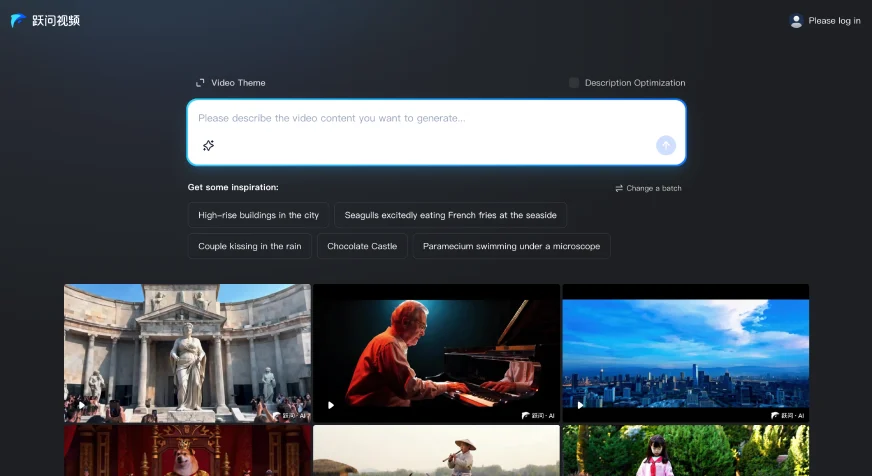
Instance of Vidoes Created by Step-Video-T2V
Listed below are some movies generated by this device. I’ve taken these from their official website.
Van Gogh in Paris
Immediate: “On the streets of Paris, Van Gogh is sitting outdoors a restaurant, portray an evening scene with a drafting board in his hand. The digicam is shot in a medium shot, displaying his targeted expression and fast-moving brush. The road lights and pedestrians within the background are barely blurred, utilizing a shallow depth of subject to spotlight his picture. As time passes, the sky modifications from nightfall to nighttime, and the celebrities step by step seem. The digicam slowly pulls away to see the comparability between his completed work and the actual evening scene.”
Millennium Falcon Journey
Immediate: “Within the huge universe, the Millennium Falcon in Star Wars is touring throughout the celebrities. The digicam reveals the spacecraft flying among the many stars in a distant view. The digicam rapidly follows the trajectory of the spacecraft, displaying its high-speed shuttle. Coming into the cockpit, the digicam focuses on the facial expressions of Han Solo and Chewbacca, who’re nervously working the devices. The lights on the dashboard flicker, and the background starry sky rapidly passes by outdoors the porthole.”
Conclusion
Step-Video-T2V isn’t accessible outdoors China but. As soon as it’s public, I’ll take a look at and share my overview. Nonetheless, it alerts a serious advance in China’s generative AI, proving its labs are shaping multimodal AI’s future alongside OpenAI and DeepMind. The subsequent step for video era calls for higher instruction-following, physics simulation, and richer datasets. Step-Video-T2V paves the best way for open-source video fashions, empowering international researchers and creators. China’s AI momentum suggests extra reasonable and environment friendly text-to-video improvements forward