DeepSeek AI has simply launched its extremely anticipated DeepSeek R1 reasoning fashions, setting new requirements on the earth of generative synthetic intelligence. With a give attention to reinforcement studying (RL) and an open-source ethos, DeepSeek-R1 delivers superior reasoning capabilities whereas being accessible to researchers and builders around the globe. The mannequin is ready to compete with OpenAI’s o1 mannequin and infact has outperformed the identical on a number of benchmarks. With DeepSeek R1, it certainly has made lots of people surprise if is it an finish to Open AI LLM supremacy. Let’s dive in to learn extra!
What’s DeepSeek R1?
DeepSeek-R1 is a reasoning-focused massive language mannequin (LLM) developed to boost reasoning capabilities in Generative AI methods by the strategy of superior reinforcement studying (RL) methods.
- It represents a major step towards bettering reasoning in LLMs, notably with out relying closely on supervised fine-tuning (SFT) as a preliminary step.
- Basically, DeepSeek-R1 addresses a key problem in AI: enhancing reasoning with out relying closely on supervised fine-tuning (SFT).
Modern coaching methodologies energy the fashions to deal with advanced duties like arithmetic, coding, and logic.
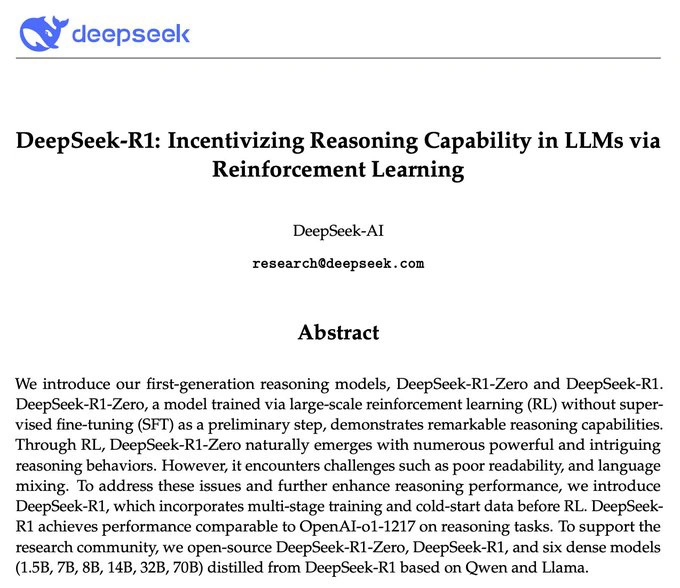
Additionally Learn: Andrej Karpathy Praises DeepSeek V3’s Frontier LLM, Educated on a $6M Finances
DeepSeek-R1: Coaching
1. Reinforcement Studying
- DeepSeek-R1-Zero is educated solely utilizing reinforcement studying (RL) with none SFT. This distinctive method incentivizes the mannequin to autonomously develop superior reasoning capabilities like self-verification, reflection, and CoT (Chain-of-Thought) reasoning.
Reward Design
- The system assigns rewards for reasoning accuracy primarily based on task-specific benchmarks.
- It additionally provides secondary rewards for structured, readable, and coherent reasoning outputs.
Rejection Sampling
- Throughout RL, a number of reasoning trajectories are generated, and the best-performing ones are chosen to information the coaching course of additional.
2. Chilly-Begin Initialization with Human-Annotated Knowledge
- For DeepSeek-R1, human-annotated examples of lengthy CoT reasoning are used to initialize the coaching pipeline. This ensures higher readability and alignment with consumer expectations.
- This step bridges the hole between pure RL coaching (which might result in fragmented or ambiguous outputs) and high-quality reasoning outputs.
3. Multi-Stage Coaching Pipeline
- Stage 1: Chilly-Begin Knowledge Pretraining: A curated dataset of human annotations primes the mannequin with fundamental reasoning buildings.
- Stage 2: Reinforcement Studying: The mannequin tackles RL duties, incomes rewards for accuracy, coherence, and alignment.
- Stage 3: Positive-Tuning with Rejection Sampling: The system fine-tunes outputs from RL and reinforces one of the best reasoning patterns.
4. Distillation
- Bigger fashions educated with this pipeline are distilled into smaller variations, sustaining reasoning efficiency whereas drastically decreasing computational prices.
- Distilled fashions inherit the capabilities of bigger counterparts, equivalent to DeepSeek-R1, with out vital efficiency degradation.
DeepSeek R1: Fashions
DeepSeek R1 comes with two core and 6 distilled fashions.
Core Fashions
DeepSeek-R1-Zero
Educated solely by reinforcement studying (RL) on a base mannequin, with none supervised fine-tuning.Demonstrates superior reasoning behaviors like self-verification and reflection, attaining notable outcomes on benchmarks equivalent to:
Challenges: Struggles with readability and language mixing resulting from a scarcity of cold-start knowledge and structured fine-tuning.
DeepSeek-R1
Builds upon DeepSeek-R1-Zero by incorporating cold-start knowledge (human-annotated lengthy chain-of-thought (CoT) examples) for enhanced initialization.Introduces multi-stage coaching, together with reasoning-oriented RL and rejection sampling for higher alignment with human preferences.
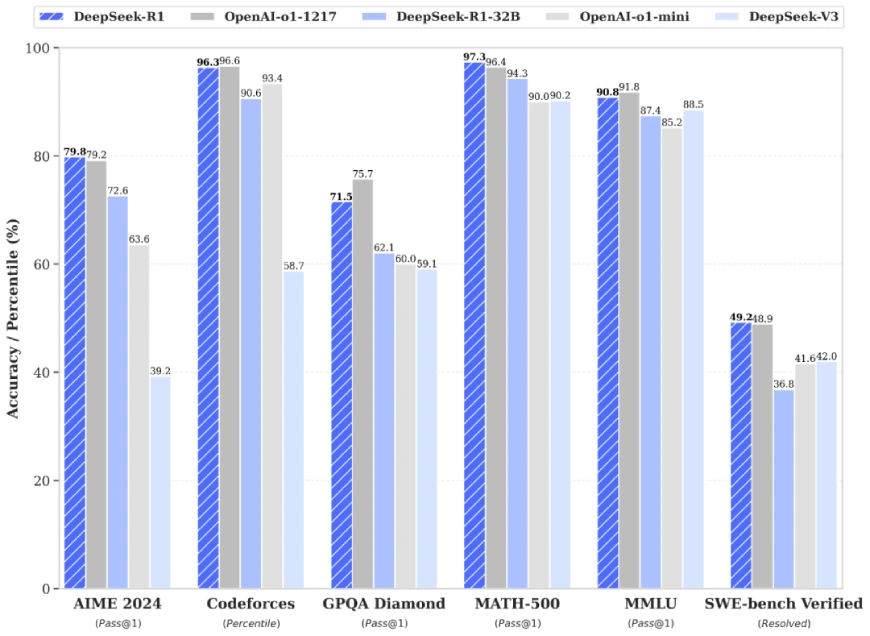
Competes straight with OpenAI’s o1-1217, attaining:
- AIME 2024: Go@1 rating of 79.8%, marginally outperforming o1-1217.
- MATH-500: Go@1 rating of 97.3%, on par with o1-1217.
Excels in knowledge-intensive and STEM-related duties, in addition to coding challenges.
Distilled Fashions
In a groundbreaking transfer, DeepSeek-AI has additionally launched distilled variations of the R1 mannequin, guaranteeing that smaller, computationally environment friendly fashions inherit the reasoning prowess of their bigger counterparts. These distilled fashions embody:
These smaller fashions outperform open-source rivals like QwQ-32B-Preview whereas competing successfully with proprietary fashions like OpenAI’s o1-mini.
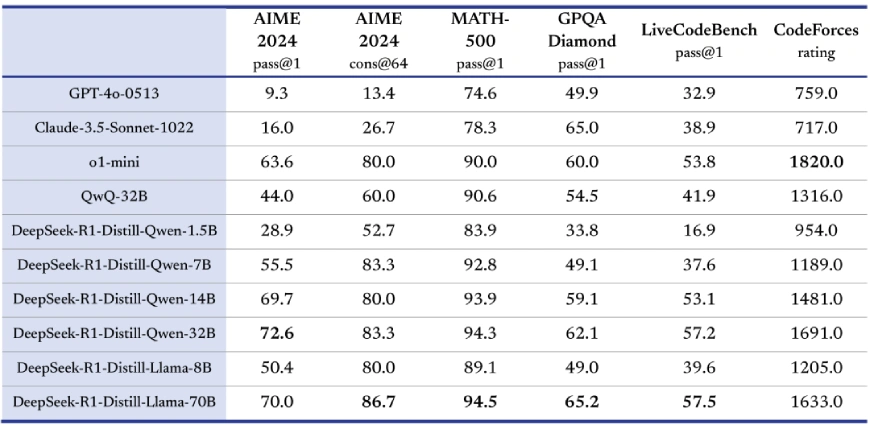
DeepSeek R1: Key Highlights
DeepSeek-R1 fashions are engineered to rival a few of the most superior LLMs within the business. On benchmarks equivalent to AIME 2024, MATH-500, and Codeforces, DeepSeek-R1 demonstrates aggressive or superior efficiency when in comparison with OpenAI’s o1-1217 and Anthropic’s Claude Sonnet 3:
- AIME 2024 (Go@1)
- MATH-500
- Codeforces
Along with its excessive efficiency, DeepSeek-R1’s open-source availability positions it as a cheap different to proprietary fashions, decreasing limitations to adoption.
The right way to Entry R1?
Net Entry
Not like OpenAI’s o1 for which it’s important to pay a premium value, DeepSeek has made its R1 mannequin free for everybody to strive of their chat interface.
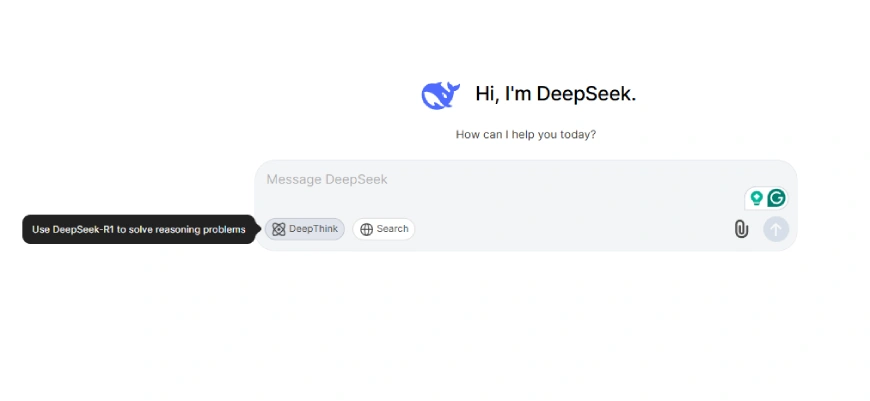
API Entry
You’ll be able to entry its API right here: https://api-docs.deepseek.com/
With a base enter value as little as $0.14 per million tokens for cache hits, DeepSeek-R1 is considerably extra reasonably priced than many proprietary fashions (e.g., OpenAI GPT-4 enter prices begin at $0.03 per 1K tokens or $30 per million tokens).
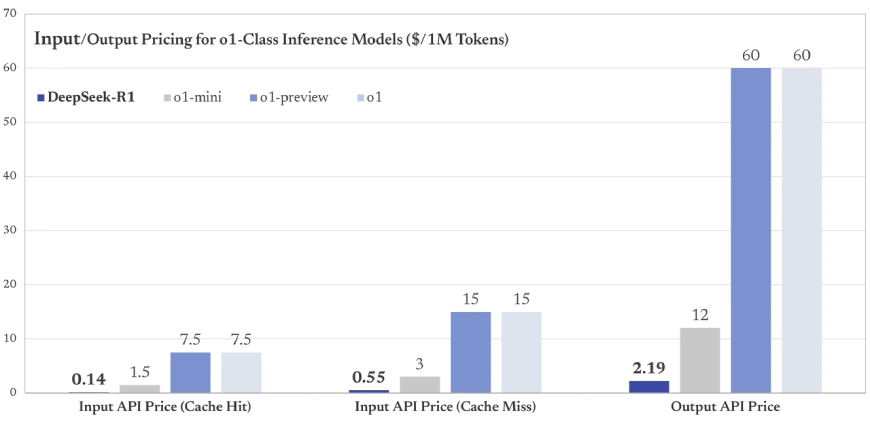
Functions
- STEM Schooling: Excelling in math-intensive benchmarks, these fashions can help educators and college students in fixing advanced issues.
- Coding and Software program Growth: With excessive efficiency on platforms like Codeforces and LiveCodeBench, DeepSeek-R1 is right for aiding builders.
- Normal Information Duties: Its prowess in benchmarks like GPQA Diamond positions it as a strong device for fact-based reasoning.
Additionally Learn:
Finish Notice
By open-sourcing the DeepSeek-R1 household of fashions, together with the distilled variations, DeepSeek-AI is making high-quality reasoning capabilities accessible to the broader AI group. This initiative not solely democratizes entry but in addition fosters collaboration and innovation.
Because the AI panorama evolves, DeepSeek-R1 stands out as a beacon of progress, bridging the hole between open-source flexibility and state-of-the-art efficiency. With its potential to reshape reasoning duties throughout industries, DeepSeek-AI is poised to grow to be a key participant within the AI revolution.
Keep tuned for extra updates on Analytics Vidhya Information!