At a time when main AI corporations make the slightest of an replace of their interface – a “breakthrough” second; Meta AI has redefined this tradition. Launching not one however THREE fashions on the identical day underneath the “Llama 4 herd”. Llama 4 consists of three fashions: Scout, Maverick, and Behemoth. Every is designed with a particular aim in thoughts—from light-weight deployment to enterprise-level reasoning. And the perfect half? Two of them can be found to the general public proper now. In a time when corporations like OpenAI, Google, and X.com are constructing more and more massive however closed fashions, Meta has chosen a unique route: making highly effective AI open and accessible. On this weblog, we are going to discover the capabilities, options, and efficiency of the three newest Llama 4 fashions: Scout, Maverick, and Behemoth!
The Llama 4 Fashions: Scout, Maverick, and Behemoth
Meta’s Llama 4: Scout, Maverick, and Behemoth fashions are a gaggle of extremely environment friendly, open-source & multi-modal fashions. Infact, Llama 4 Maverick crossed the 1400 benchmark on the LMarena, beating fashions like GPT 4o, DeepSeek V3, Gemini 2.0 Flash, and extra! Equally notable is the ten million token context size supported by these fashions which is the longest of any open-weight LLM so far. Let’s take a look at every of those fashions intimately.
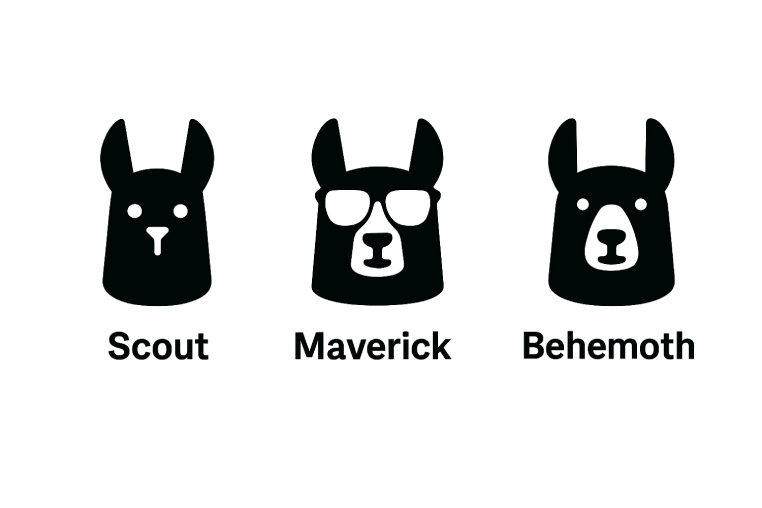
Llama 4 Scout: Small, Quick, and Sensible
Scout is probably the most environment friendly mannequin within the Llama 4 household. It’s a quick and light-weight mannequin, superb for builders and researchers who don’t have entry to massive GPU clusters.
Key Options of Llama 4 Scout:
- Structure: Scout makes use of a Combination of Specialists (MoE) structure with 16 consultants, activating solely 2 at a time, which leads to 17B energetic parameters from a complete of 109B. It helps a ten million token context window.
- Effectivity: The mannequin runs effectively on a single H100 GPU utilizing Int4 quantization, making it an reasonably priced high-performance possibility.
- Efficiency: Scout outperforms peer fashions akin to Gemma 3, Gemini 2.0 Flash-Lite, and Mistral 3.1 in benchmark checks.
- Coaching: It has been pre-trained in 200 languages 100 of which embrace over a billion tokens every and educated on various picture and video knowledge, supporting as much as 8 pictures in a single immediate.
- Utility: Because of superior picture area grounding, it delivers exact visible reasoning. This makes it superb for functions akin to long-context reminiscence chatbots, code summarization instruments, instructional Q&A bots, and assistants optimized for cell or embedded programs.
Llama 4 Maverick: Sturdy and Dependable
Maverick is the flagship open-weight mannequin. It’s designed for superior reasoning, coding, and multimodal functions. Whereas it’s extra highly effective than Scout, it maintains effectivity utilizing the identical MoE technique.
Key Options of Llama 4 Maverick:
- Structure: Maverick makes use of a Combination of Specialists structure with 128 routed consultants and a shared professional, activating solely 17B parameters out of a complete of 400B throughout inference. It’s educated utilizing an early fusion of textual content and picture inputs and helps as much as 8 picture inputs.
- Effectivity: The mannequin runs effectively on a single H100 DGX host or might be scaled throughout GPUs.
- Efficiency: It achieves an ELO rating of 1417 on the LMSYS Chatbot Area, outperforming GPT-4o and Gemini 2.0 Flash, whereas additionally matching DeepSeek v3.1 in reasoning, coding, and multilingual capabilities.
- Coaching: Maverick was constructed with cutting-edge methods akin to MetaP hyperparameter scaling, FP8 precision coaching, and a 30 trillion token dataset. It delivers sturdy picture understanding, multilingual reasoning, and cost-efficient efficiency that surpasses the Llama 3.3 70B mannequin.
- Functions: Its strengths make it superb for AI pair programming, enterprise-level doc understanding, and academic tutoring programs.
Llama 4 Behemoth: The Trainer Mannequin
Behemoth is Meta’s largest mannequin so far. It isn’t out there for public use, however it performed a significant position in serving to Scout and Maverick develop into what they’re in the present day.
Key Options of Llama 4 Behemoth:
- Structure: Behemoth is Meta’s largest and strongest mannequin, utilizing a Combination of Specialists structure with 16 consultants and activating 288B parameters out of almost 2 trillion throughout inference. It’s natively multimodal and excels in reasoning, math, and vision-language duties.
- Efficiency: Behemoth persistently outperforms GPT-4.5, Claude Sonnet 3.7, and Gemini 2.0 Professional on STEM benchmarks like MATH-500, GPQA Diamond, and BIG-bench.
- Position: It performs a key position as a trainer mannequin, guiding Scout and Maverick by way of co-distillation with a novel loss operate that balances smooth and laborious supervision.
- Coaching: The mannequin was educated utilizing FP8 precision, optimized MoE parallelism for 10x pace good points over Llama 3, and a brand new reinforcement studying technique. This included laborious immediate sampling, multi-capability batch development, and sampling from a wide range of system directions.
Although not publicly out there, Behemoth serves as Meta’s gold customary for analysis and inner distillation.
Entry Llama 4 Fashions:
You can begin utilizing Llama 4 in the present day by way of a number of easy-to-use platforms, relying in your objectives—whether or not it’s analysis, utility improvement, or simply testing out capabilities.
- llama.meta.com: That is Meta’s official hub for Llama fashions. It consists of mannequin playing cards, papers, technical documentation, and entry to the open weights for each Scout and Maverick. Builders can obtain the fashions and run them domestically or within the cloud.
- Hugging Face: Hugging Face hosts the ready-to-use variations of Llama 4. You’ll be able to take a look at fashions immediately within the browser utilizing inference endpoints or deploy them through the Transformers library. Integration with frequent instruments like Gradio and Streamlit can be supported.
- Meta Apps: The Llama 4 fashions additionally energy Meta’s AI assistant out there in WhatsApp, Instagram, Messenger, and Fb. This enables customers to expertise the fashions in real-world conversations, immediately inside their on a regular basis apps.
- Internet web page: You’ll be able to immediately entry the most recent Llama 4 fashions utilizing the net interface.
Llama 4 Fashions: Let’s Attempt!
It’s tremendous straightforward to attempt the most recent Llama 4 fashions throughout any of Meta’s apps or the net interface. Though it isn’t particularly talked about in any of these relating to which fashions: Out of Scout, Maverick, or Behemoth it’s utilizing within the background. As of now, Meta AI hasn’t supplied a selection to decide on the mannequin that you simply want to work with on its apps or interface. Nonetheless; I’ll take a look at the Llama 4 mannequin for 2 duties: Inventive Planning, Coding and Picture Technology.
Job 1: Inventive Planning
Immediate: “Create a Social Media content material technique for a Shoe Model – Soles to assist them have interaction with the Gen z viewers”
Output:
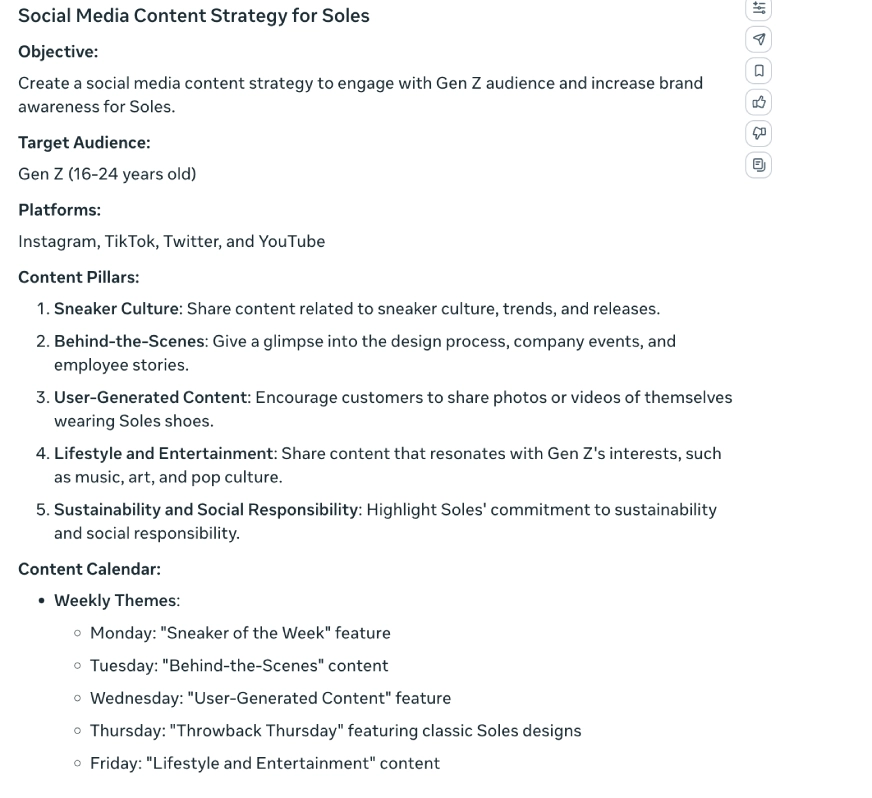
- Llama 4 fashions are very quick! The mannequin shortly maps put an in depth but concise plan for the social media technique.
- Within the internet interface, you may’t at the moment add any recordsdata or pictures.
- Additionally, it doesn’t help internet search or canvas options but.
Job 2: Coding
Immediate:” Write a python program that exhibits a ball bouncing inside a spinning pentagon, following the legal guidelines of Physics, rising its pace each time it bounces off an edge.”
Output:
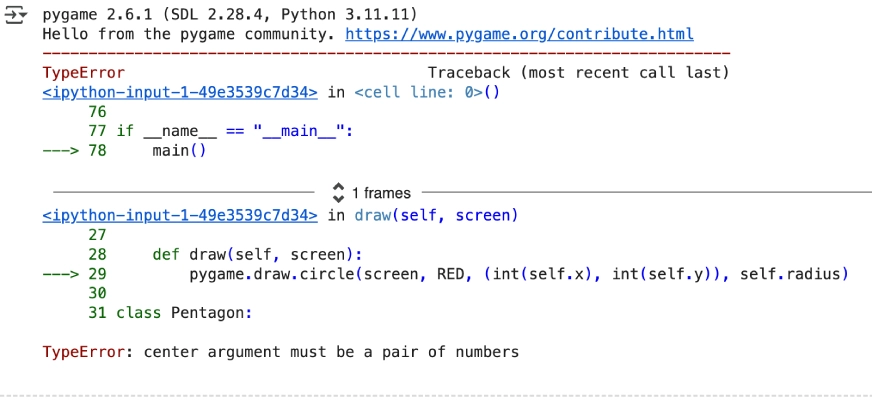
- The code it generated had errors.
- The mannequin shortly processes the requirement however but just isn’t nice relating to accuracy.
Job 3: Picture Technology
Immediate: “create a picture of an individual engaged on a laptop computer with a doc open within the laptop computer with the title “llama 4”, the picture needs to be taken in a approach the display of the particular person is seen, the desk on which the laptop computer is saved has a espresso mug and a plant”
Output:
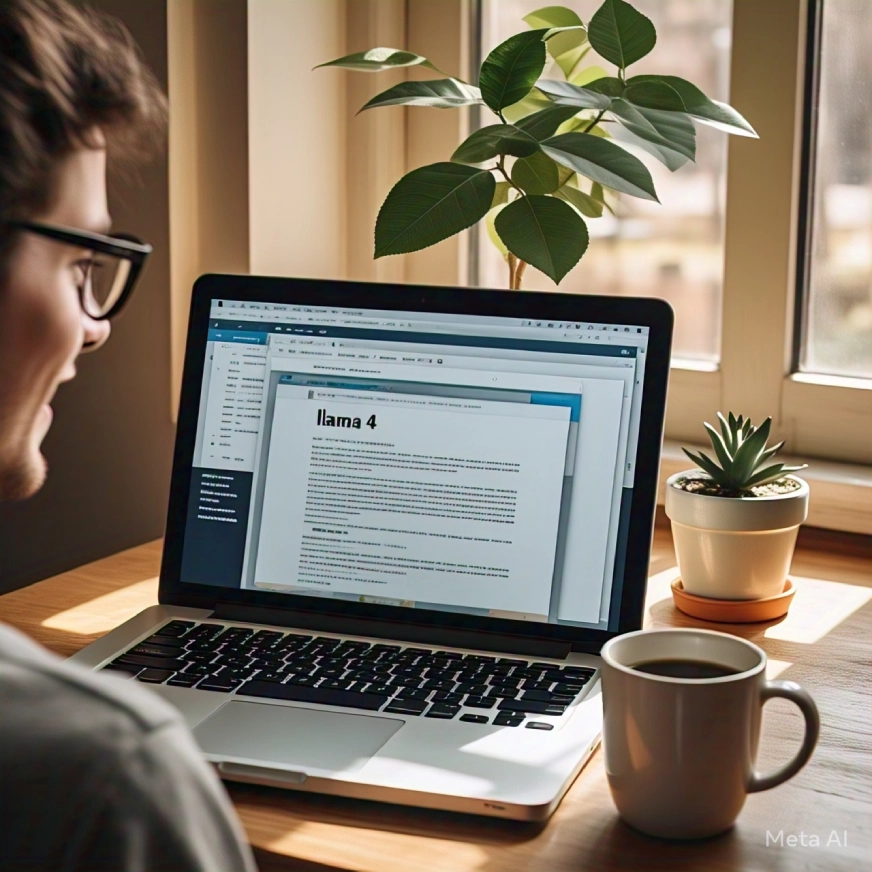
- It generated 4 pictures! Out of these, I discovered the above picture to be the perfect.
- You additionally get the choice to “Edit” and “Animate” the photographs that you’ve generated.
- Enhancing means that you can rework sure sections of a picture whereas Animating means that you can create a gif of the picture.
Coaching and Put up-Coaching: Llama 4 Fashions
Meta used a structured two-step course of: pre-training and post-training, incorporating new methods for higher efficiency, scalability, and effectivity. Let’s break down the entire course of:
Pre-Coaching Llama 4 Fashions:
Pre-training is the muse for a mannequin’s data and talent. Meta launched a number of improvements on this stage:
- Multimodal Information: Llama 4 fashions had been educated on over 30 trillion tokens from various textual content, picture, and video datasets. They’re natively multimodal, that means they deal with each language and imaginative and prescient from the beginning.
- Combination of Specialists (MoE): Solely a subset of the mannequin’s whole parameters is energetic throughout every inference. This selective routing permits large fashions like Maverick (400B whole parameters) and Behemoth (~2T) to be extra environment friendly.
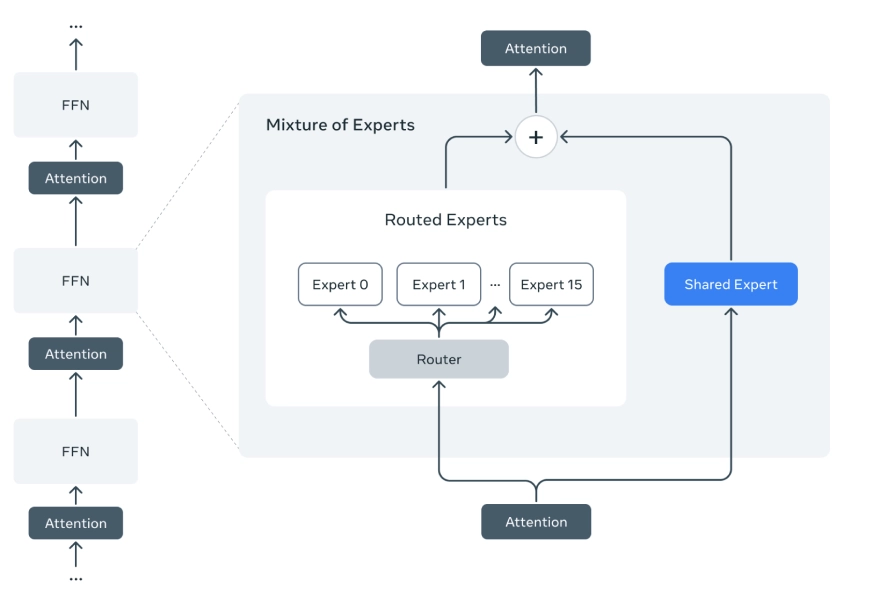
- Early Fusion Structure: Textual content and imaginative and prescient inputs are collectively educated utilizing early fusion, integrating each right into a shared mannequin spine.
- MetaP Hyperparameter Tuning: This new approach lets Meta set per-layer studying charges and initialization scales that switch effectively throughout mannequin sizes and coaching configurations.
- FP8 Precision: All fashions use FP8 for coaching, which will increase computing effectivity with out sacrificing mannequin high quality.
- iRoPE Structure: A brand new strategy utilizing interleaved consideration layers with out positional embeddings and inference-time temperature scaling, serving to Scout generalize to extraordinarily lengthy inputs (as much as 10M tokens).
Put up-Coaching Llama 4 Fashions:
As soon as the bottom fashions had been educated, they had been fine-tuned utilizing a fastidiously crafted sequence:
- Light-weight Supervised Nice-Tuning (SFT): Meta filtered out straightforward prompts utilizing Llama fashions as judges and solely used tougher examples to fine-tune efficiency on complicated reasoning duties.
- On-line Reinforcement Studying (RL): They applied steady RL coaching utilizing laborious prompts, adaptive filtering, and curriculum design to take care of reasoning, coding, and conversational capabilities.
- Direct Desire Optimization (DPO): After RL, light-weight DPO was utilized to fine-tune particular nook instances and response high quality, balancing helpfulness and security.
- Behemoth Codistillation: Behemoth acted as a trainer by producing outputs for coaching Scout and Maverick. Meta even launched a novel loss operate to dynamically stability smooth and laborious supervision targets.
Collectively, these steps produced fashions that aren’t simply massive—however deeply optimized, safer, and extra succesful throughout various duties.
Benchmark Efficiency
Meta has shared detailed benchmark outcomes for all three Llama 4 fashions, reflecting how every performs primarily based on its design objectives and parameter sizes. Additionally they outperform main fashions in a number of newly launched benchmarks which can be significantly difficult and complete.
Llama 4 Scout: Benchmarks
Scout, regardless of being the smallest within the household, performs remarkably effectively in efficiency-focused evaluations:
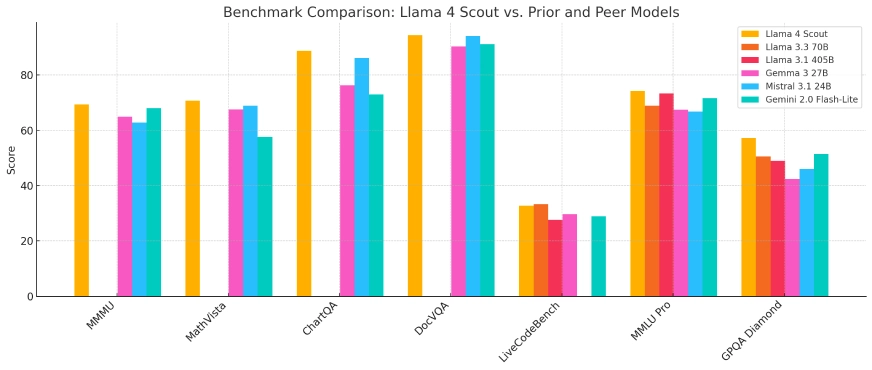
- ARC (AI2 Reasoning Problem): Scores competitively amongst fashions in its measurement class, significantly in commonsense reasoning.
- MMLU Lite: Performs reliably on duties like historical past, fundamental science, and logical reasoning.
- Inference Pace: Exceptionally quick, even on a single H100 GPU, with low latency responses in QA and chatbot duties.
- Code Technology: Performs effectively for easy to intermediate programming duties, making it helpful for instructional coding assistants.
- Needle-in-a-Haystack (NiH): Achieves near-perfect retrieval in long-context duties with as much as 10M tokens of textual content or 20 hours of video, demonstrating unmatched long-term reminiscence.
Llama 4 Maverick: Benchmarks
Maverick is constructed for efficiency, and it delivers throughout the board:
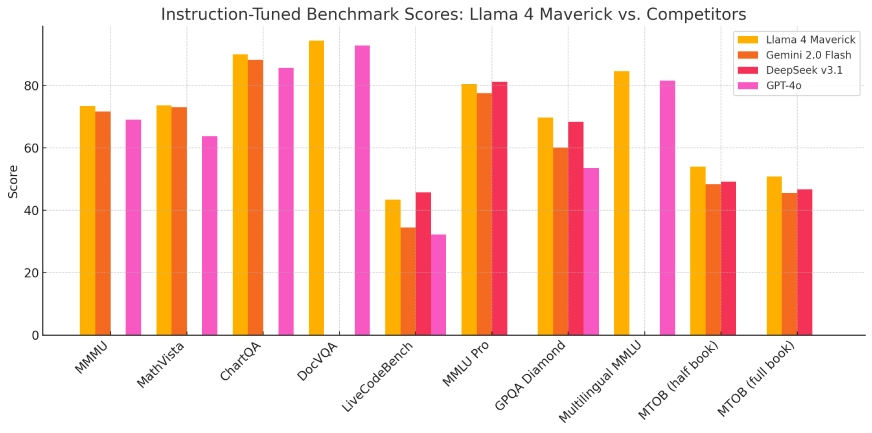
- MMLU (Multitask Language Understanding): Outperforms GPT-4o, Gemini 1.5 Flash, and Claude 3 Sonnet in knowledge-intensive duties.
- HumanEval (Code Technology): Matches or surpasses GPT-4 in producing useful code and fixing algorithmic issues.
- DROP (Discrete Reasoning Over Paragraphs): Reveals sturdy contextual understanding and numerical reasoning.
- VQAv2 (Visible Query Answering): Excels at answering image-based queries precisely, showcasing Maverick’s sturdy vision-language skills.
- Needle-in-a-Haystack (NiH): Efficiently retrieves hidden info throughout lengthy paperwork as much as 1M tokens, with near-perfect accuracy and just a few misses at excessive context depths.
Llama 4 Behemoth: Benchmarks
Behemoth just isn’t out there to the general public however serves as Meta’s strongest analysis benchmark. It’s used to distill and information different fashions:
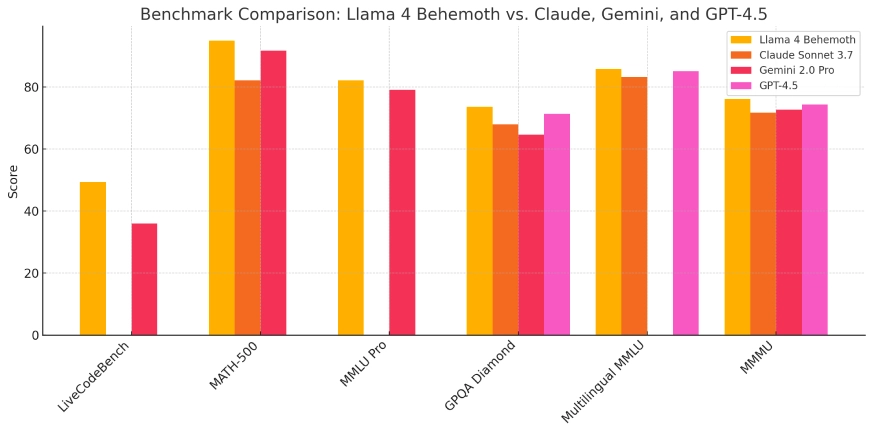
- Inside STEM Benchmarks: Tops inner Meta checks in science, math, and reasoning.
- SuperGLUE and BIG-bench: Achieves high scores internally, reflecting cutting-edge language modeling functionality.
- Imaginative and prescient-Language Integration: Reveals distinctive efficiency on duties requiring mixed textual content and picture understanding, typically surpassing all recognized public fashions.
These benchmarks present that every mannequin is well-optimized for its position: Scout for pace and effectivity, Maverick for energy and general-purpose duties, and Behemoth as a research-grade trainer mannequin for distillation and analysis.
Evaluating the Llama 4 Fashions: Scout, Maverick & Behemoth
Whereas all of the three fashions include their very own options, here’s a transient abstract that may provide help to discover the appropriate Llama 4 mannequin in your activity:
Mannequin | Whole Params | Energetic Params | Specialists | Context Size | Runs on | Public Entry | Excellent For |
Scout | 109B | 17B | 16 | 10M tokens | Single H100 | ✅ | Gentle AI duties, lengthy reminiscence apps |
Maverick | 400B | 17B | 128 | Unlisted | Single or Multi-GPU | ✅ | Analysis, coding, enterprise use |
Behemoth | ~2T | 288B | 16 | Unlisted | Inside infra | ❌ | Inside distillation + benchmarks |
Conclusion:
With the Llama 4 launch, Meta is doing extra than simply maintaining it’s setting a brand new customary.
These fashions are highly effective, environment friendly, and open. Builders don’t want enormous budgets to work with top-tier AI anymore. From small companies to massive enterprises, from school rooms to analysis labs Llama 4 places cutting-edge AI into everybody’s palms. Within the rising world of AI, openness is now not a aspect story; it’s the longer term. And Meta simply gave it a robust voice.
Login to proceed studying and revel in expert-curated content material.