In comparison with robotic techniques, people are wonderful navigators of the bodily world. Bodily processes apart, this largely comes all the way down to innate cognitive skills nonetheless missing in most robotics:
- The flexibility to localize landmarks at various ontological ranges, reminiscent of a “ebook” being “on a shelf” or “in the lounge”
- Having the ability to rapidly decide whether or not there’s a navigable path between two factors primarily based on the atmosphere structure
Early robotic navigation techniques relied on primary line-following techniques. These ultimately advanced into navigation primarily based on visible notion, offered by cameras or LiDAR, to assemble geometric maps. In a while, Simultaneous Localization and Mapping (SLAM) techniques have been built-in to supply the flexibility to plan routes by way of environments.
About us: Viso Suite is our end-to-end laptop imaginative and prescient infrastructure for enterprises. By offering a single location to develop, deploy, handle, and safe the applying growth course of, Viso Suite omits the necessity for level options. Enterprise groups can enhance productiveness and decrease operation prices with full-scale options to speed up the ML pipeline. Ebook a demo with our crew of consultants to be taught extra.
Multimodal Robotic Navigation – The place Are We Now?
More moderen makes an attempt to endow robotics with the identical capabilities have centered round constructing geometric maps for path planning and parsing objectives from pure language instructions. Nevertheless, this method struggles relating to generalizing for brand spanking new or beforehand unseen directions. To not point out environments that change dynamically or are ambiguous in a roundabout way.
Moreover, studying strategies immediately optimize navigation insurance policies primarily based on end-to-end language instructions. Whereas this methodology just isn’t inherently unhealthy, it does require huge quantities of knowledge to coach fashions.
Present Synthetic Intelligence (AI) and deep studying fashions are adept at matching object photos to pure language descriptions by leveraging coaching on internet-scale knowledge. Nevertheless, this functionality doesn’t translate properly to mapping the environments containing the mentioned objects.
New analysis goals to combine multimodal inputs to boost robotic navigation in complicated environments. As a substitute of basing route planning on one-dimensional visible enter, these techniques mix visible, audio, and language cues. This enables for making a richer context and bettering situational consciousness.
Introducing AVLMaps and VLMaps – A New Paradigm for Robotic Navigation?
One doubtlessly groundbreaking space of research on this subject pertains to so-called VLMaps (Visible Language Maps) and AVLMaps (Audio Visible Language Maps). The latest papers “Visible Language Maps for Robotic Navigation” and “Audio Visible Language Maps for Robotic Navigation” by Chenguang Huang and co. discover the prospect of utilizing these fashions for robotic navigation in nice element.
VLMaps immediately fuses visual-language options from pre-trained fashions with 3D reconstructions of the bodily atmosphere. This permits exact spatial localization of navigation objectives anchored in pure language instructions. It might additionally localize landmarks and spatial references for landmarks.
The principle benefit is that this enables for zero-shot spatial aim navigation with out further knowledge assortment or finetuning.
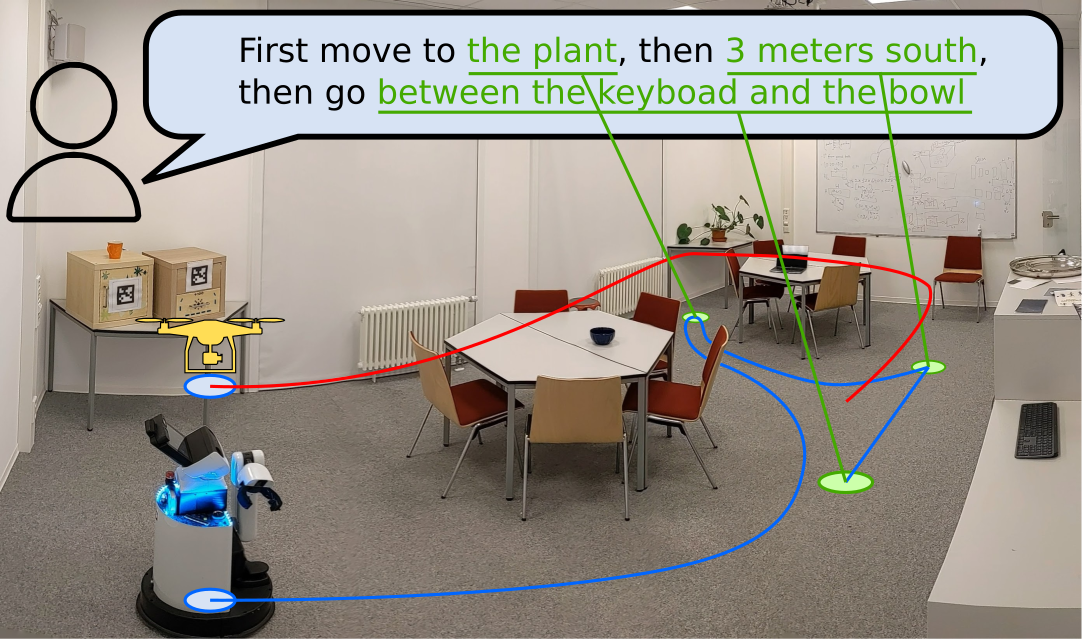
This method permits for extra correct execution of complicated navigational duties and the sharing of those maps with totally different robotic techniques.
AVLMaps are primarily based on the identical method but in addition incorporate audio cues to assemble a 3D voxel grid utilizing pre-trained multimodal fashions. This makes zero-shot multimodal aim navigation doable by indexing landmarks utilizing textual, picture, and audio inputs. For instance, this might enable a robotic to hold out a navigation aim reminiscent of “go to the desk the place the beeping sound is coming from.”
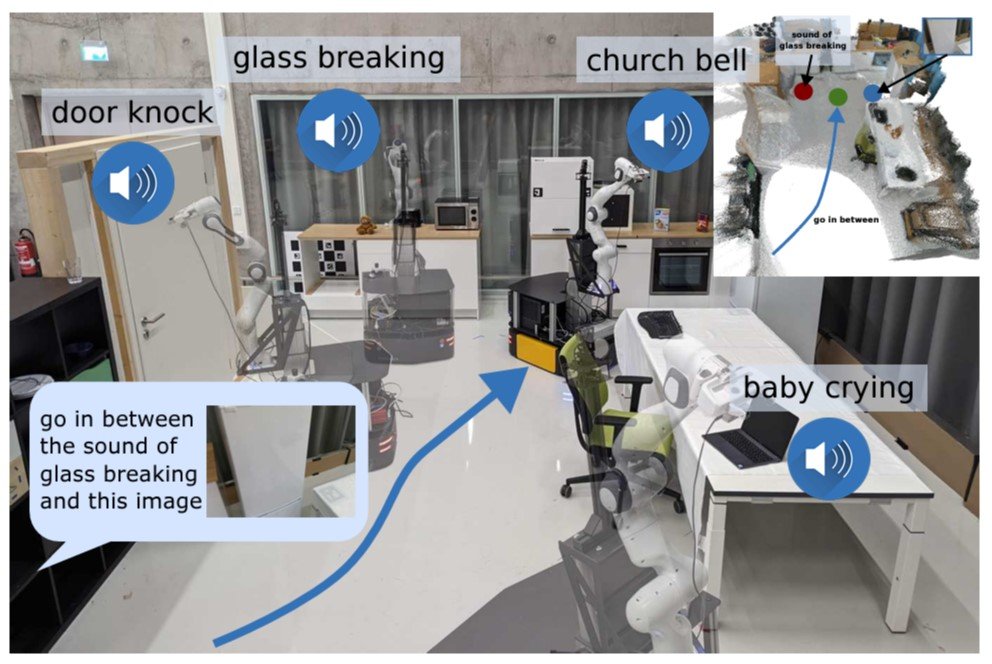
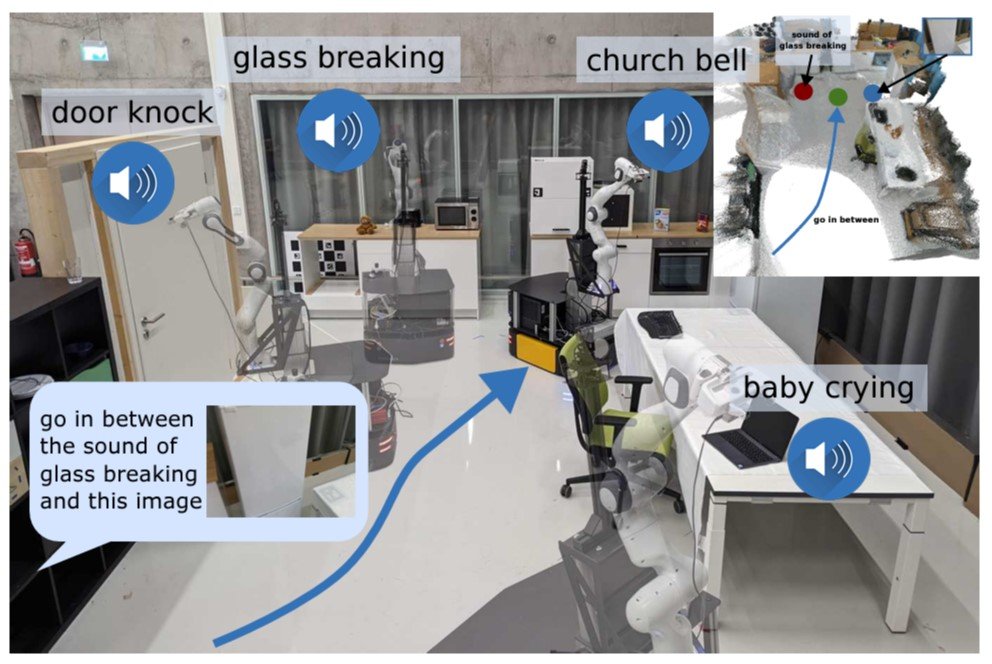
Audio enter can enrich the system’s world notion and assist disambiguate objectives in environments with a number of potential targets.
VLMaps: Integrating Visible-Language Options with Spatial Mapping
Associated work in AI and laptop imaginative and prescient has performed a pivotal function in creating VLMaps. As an example, the maturation of SLAM methods has significantly superior the flexibility to translate semantic info into 3D maps. Conventional approaches both relied on densely annotated 3D volumetric maps with 2D semantic segmentation Convolutional Neural Networks (CNNs) or object-oriented strategies to construct 3D Maps.
Whereas progress has been made in generalizing these fashions, it’s closely constrained by working on a predefined set of semantic lessons. VLMaps overcomes this limitation by creating open-vocabulary semantic maps that enable pure language indexing.
Enhancements in Imaginative and prescient and Language Navigation (VLN) have additionally led to the flexibility to be taught end-to-end insurance policies that comply with route-based directions on topological graphs of simulated environments. Nevertheless, till now, their real-world applicability has been restricted by a reliance on topological graphs and a scarcity of low-level planning capabilities. One other draw back is the necessity for big knowledge units for coaching.
For VLMaps, the researchers have been influenced by pre-trained language and imaginative and prescient fashions, reminiscent of LM-Nav and CoW (CLIP on Wheels). The latter performs zero-shot language-based object navigation by leveraging CLIP-based saliency maps. Whereas these fashions can navigate to things, they wrestle with spatial queries, reminiscent of “to the left of the chair” and “in between the TV and the couch.”
VLMaps lengthen these capabilities by supporting open-vocabulary impediment maps and sophisticated spatial language indexing. This enables navigation techniques to construct queryable scene representations for LLM-based robotic planning.
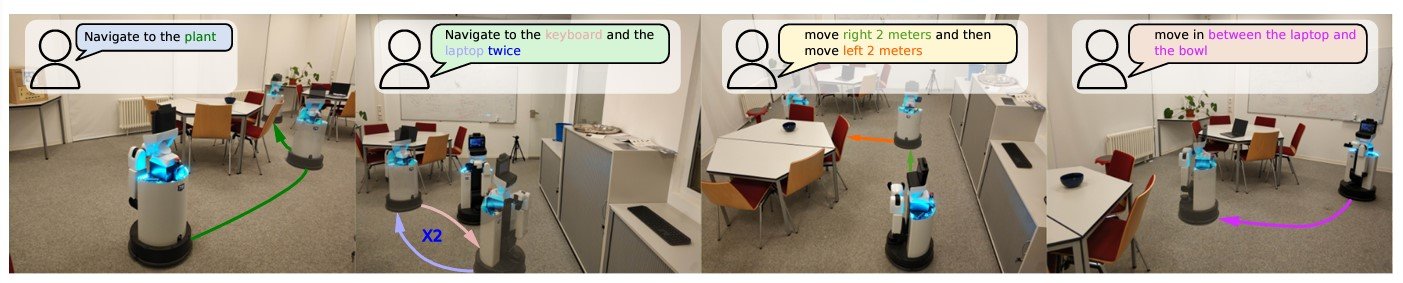
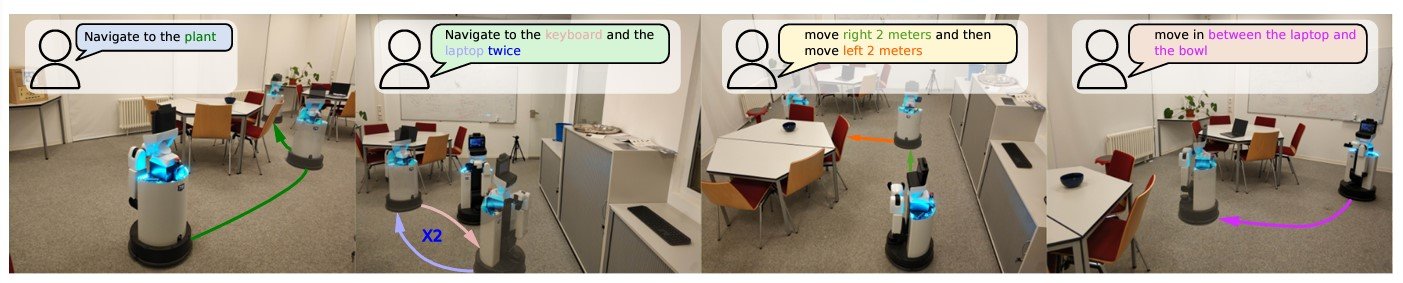
Key Parts of VLMaps
A number of key elements within the growth of VLMaps enable for constructing a spatial map illustration that localizes landmarks and spatial references primarily based on pure language.
Constructing a Visible-Language Map
VLMaps makes use of a video feed from robots mixed with commonplace exploration algorithms to construct a visual-language map. The method includes:
- Visible Characteristic Extraction: Utilizing fashions like CLIP to extract visual-language options from picture observations.
- 3D Reconstruction: Combining these options with 3D spatial knowledge to create a complete map.
- Indexing: Enabling the map to assist pure language queries, permitting for indexing and localization of landmarks.
Mathematically, suppose VV represents the visible options and LL represents the language options. In that case, their fusion could be represented as M=f(V, L)M = f(V, L), the place MM is the ensuing visual-language map.
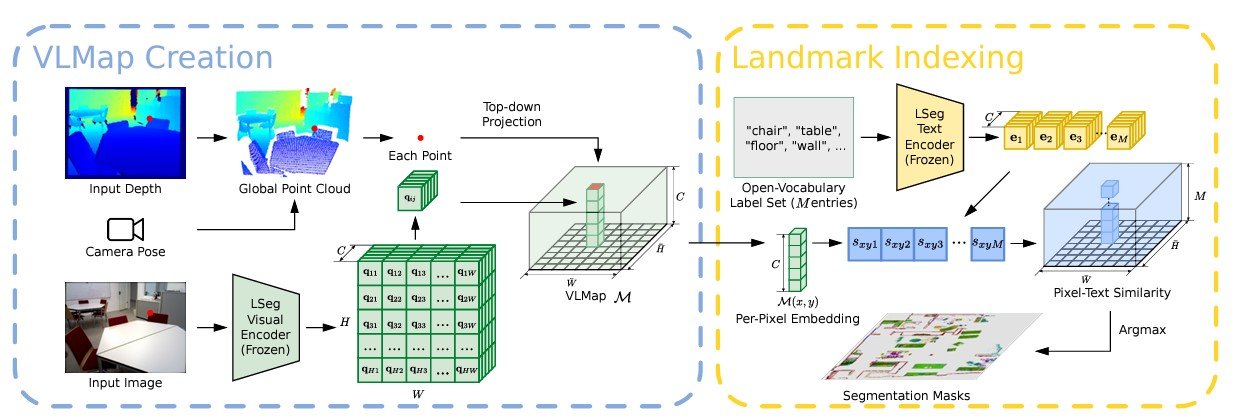
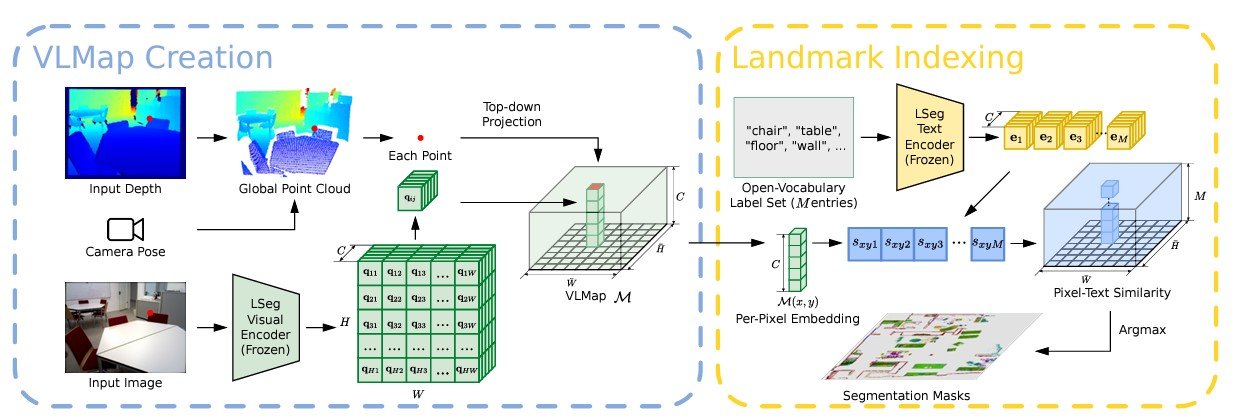
Localizing Open-Vocabulary Landmarks
To localize landmarks in VLMaps utilizing pure language, an enter language listing is outlined with representations for every class in textual content type. Examples embrace [“chair”, “sofa”, “table”] or [“furniture”, “floor”]. This listing is transformed into vector embeddings utilizing the pre-trained CLIP textual content encoder.
The map embeddings are then flattened into matrix type. The pixel-to-category similarity matrix is computed, with every component indicating the similarity worth. Making use of the argmax operator and reshaping the end result provides the ultimate segmentation map, which identifies essentially the most associated language-based class for every pixel.
Producing Open-Vocabulary Impediment Maps
Utilizing a Giant Language Mannequin (LLM), VLMap interprets instructions and breaks them into subgoals, permitting for particular directives like “in between the couch and the TV” or “three meters east of the chair.”
The LLM generates executable Python code for robots, translating high-level directions into parameterized navigation duties. For instance, instructions reminiscent of “transfer to the left facet of the counter” or “transfer between the sink and the oven” are transformed into exact navigation actions utilizing predefined capabilities.
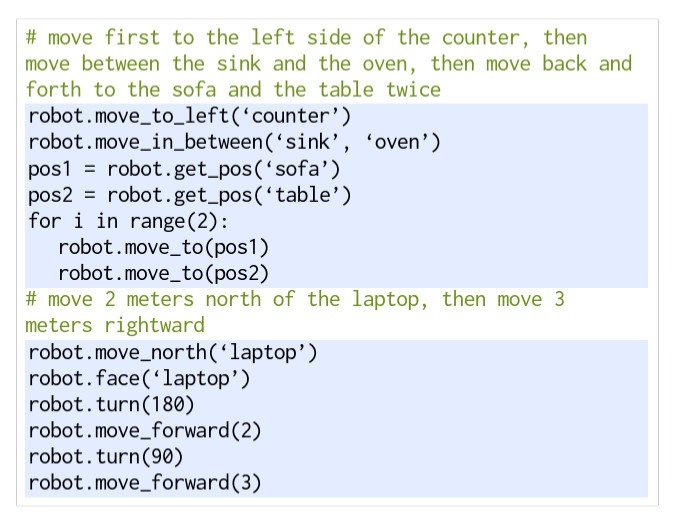
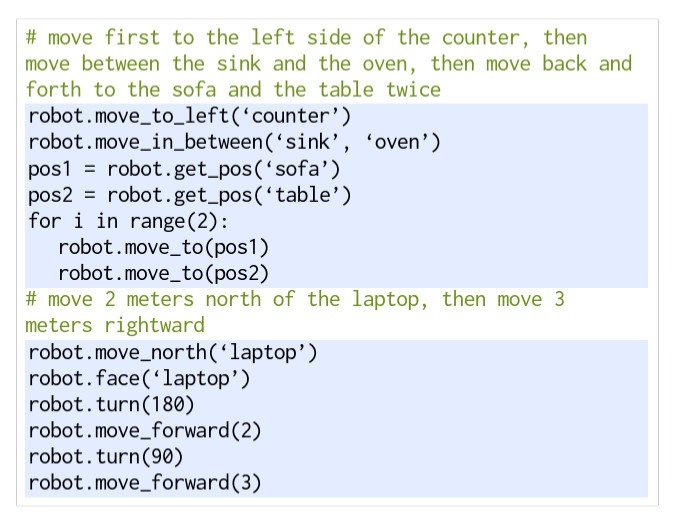
AVLMaps: Enhancing Navigation with Audio, Visible, and Language Cues
AVLMaps largely builds on the identical method utilized in creating VLMaps, however prolonged with multimodal capabilities to course of auditory enter as properly. In AVLMaps, objects could be immediately localized from pure language directions utilizing each visible and audio cues.
For testing, the robotic was additionally supplied with an RGB-D video stream and odometry info, however this time with an audio observe included.
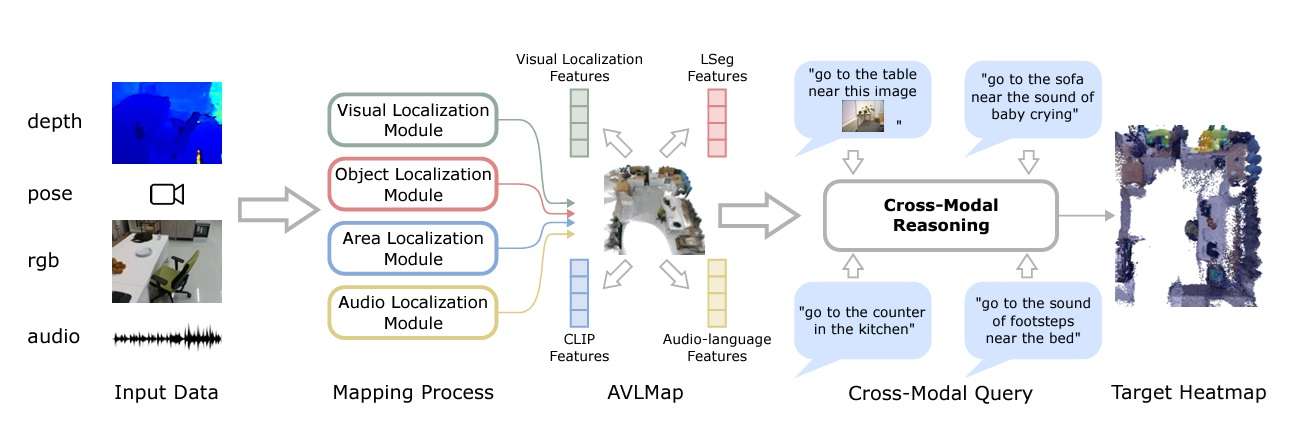
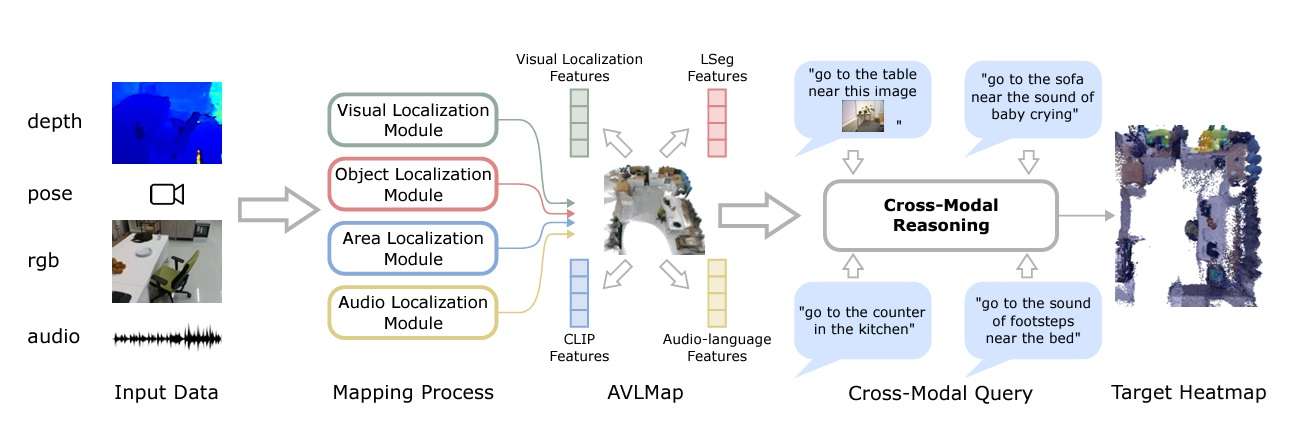
Module Sorts
In AVLMaps, the system makes use of 4 modules to construct a multimodal options database. They’re:
- Visible Localization Module: Localizes a question picture within the map utilizing a hierarchical scheme, computing each native and world descriptors within the RGB stream.
- Object Localization Module: Makes use of open-vocabulary segmentation (OpenSeg) to generate pixel-level options from the RGB picture, associating them with back-projected depth pixels in 3D reconstruction. It computes cosine similarity scores for all level and language options, choosing top-scoring factors within the map for indexing.
- Space Localization Module: The paper proposes a sparse topological CLIP options map to determine coarse visible ideas, like “kitchen space.” Additionally, utilizing cosine similarity scores, the mannequin calculates confidence scores for predicting areas.
- Audio Localization Module: Partitions an audio clip from the stream into segments utilizing silence detection. Then, it computes audio-lingual options for every utilizing AudioCLIP to provide you with matching scores for predicting areas primarily based on odometry info.
The important thing differentiator of AVLMaps is its means to disambiguate objectives by cross-referencing visible and audio options. Within the paper, that is achieved by creating heatmaps with chances for every voxel place primarily based on the space to the goal. The mannequin multiplies the outcomes from heatmaps for various modalities to foretell the goal with the best chances.
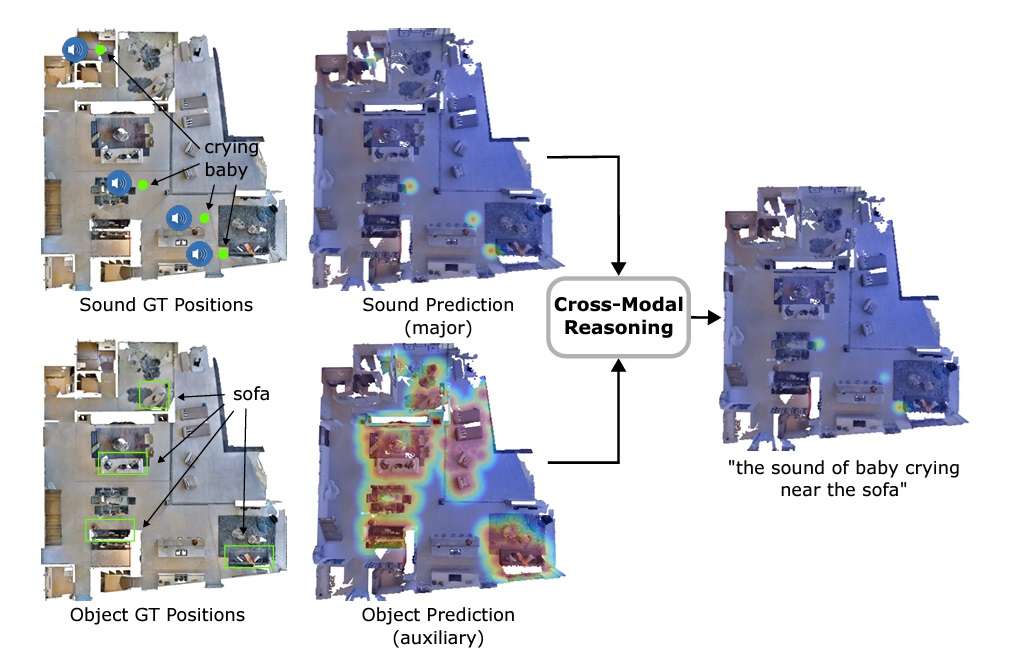
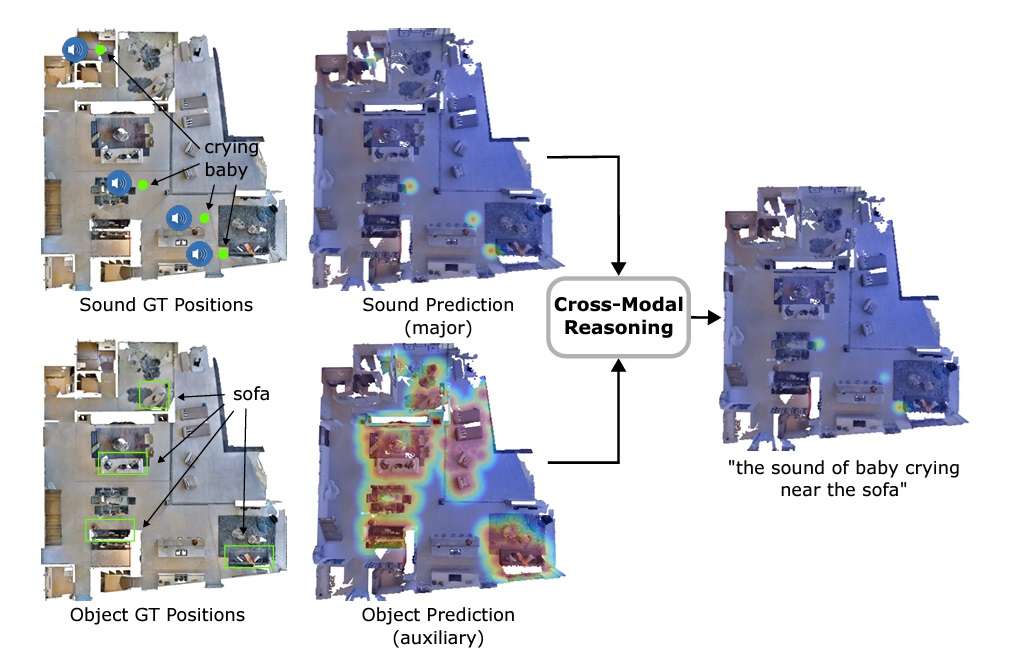
VLMaps and AVLMaps vs. Different Strategies for Robotic Navigation
Experimental outcomes present the promise of using methods like VLMaps for robotic navigation. Wanting on the object, numerous fashions have been generated for the thing kind “chair,” for instance, it’s clear that VLMaps is extra discerning in its predictions.
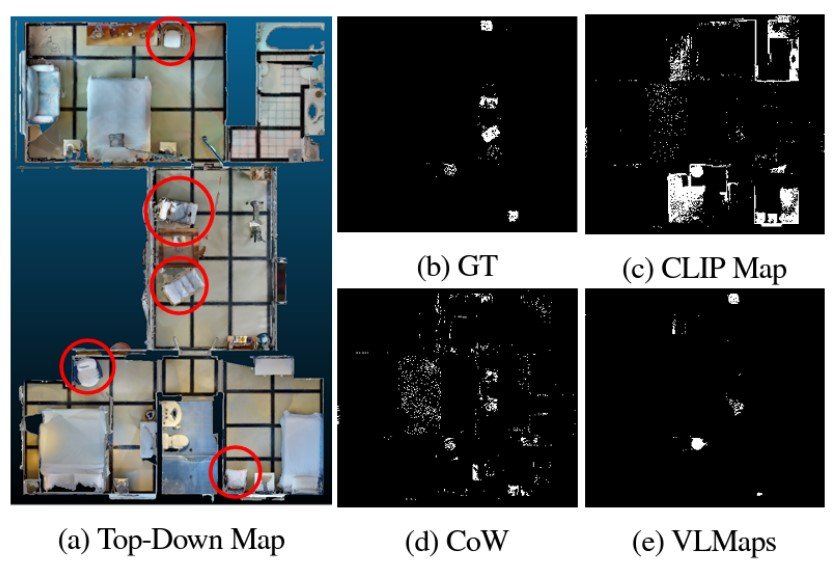
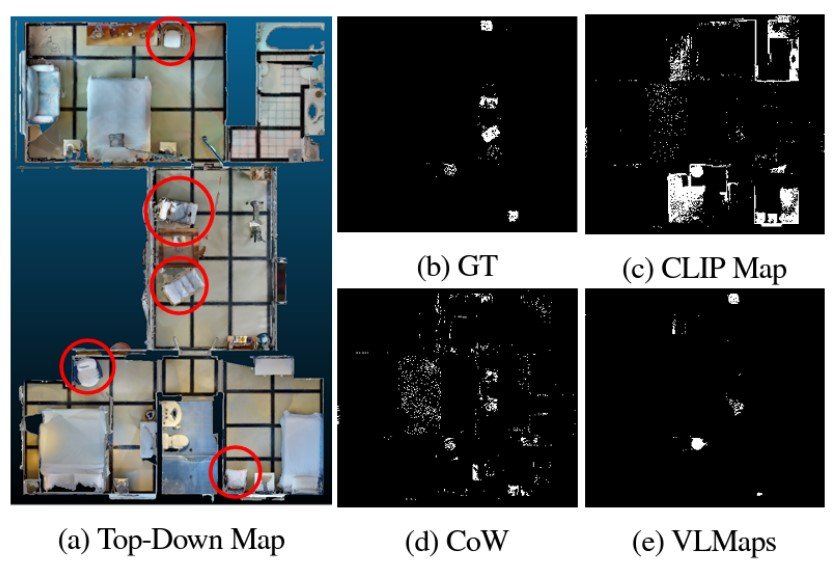
In multi-object navigation, VLMaps considerably outperformed standard fashions. That is largely as a result of VLMaps don’t endure from producing as many false positives as the opposite strategies.
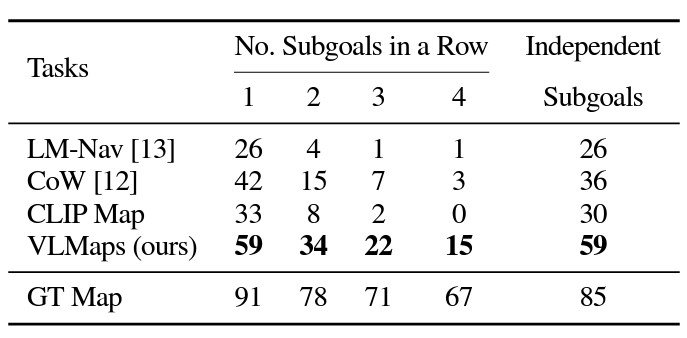
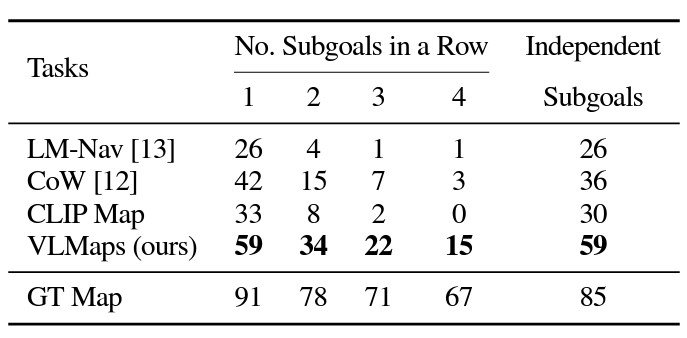
VLMaps additionally achieves a lot larger zero-shot spatial aim navigation success charges than the opposite open-vocabulary zero-shot navigation baseline options.
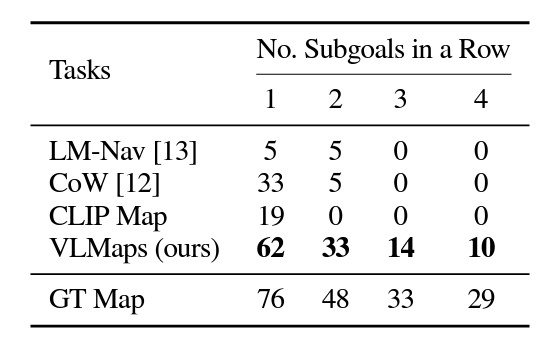
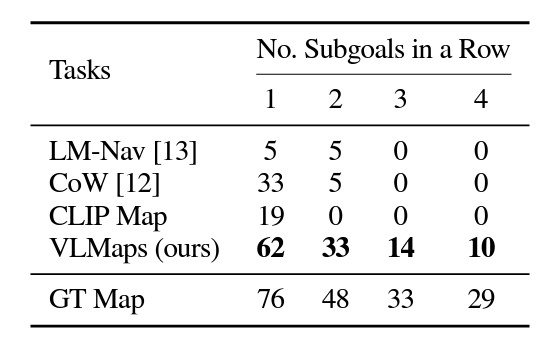
One other space the place VLMaps reveals promising outcomes is in cross-embodiment navigation to optimize route planning. On this case, VLMaps generated totally different impediment maps for robotic embodiments, a ground-based LoCoBot, and a flying drone. When supplied with a drone map, the drone considerably improved its efficiency by creating navigation maps to fly over obstacles. This reveals VLMap’s effectivity at each 2D and 3D spatial navigation.
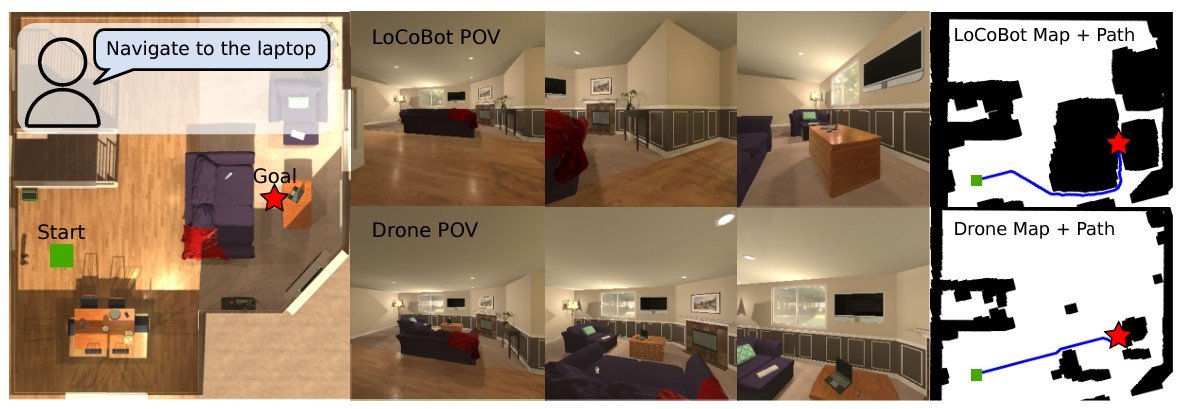
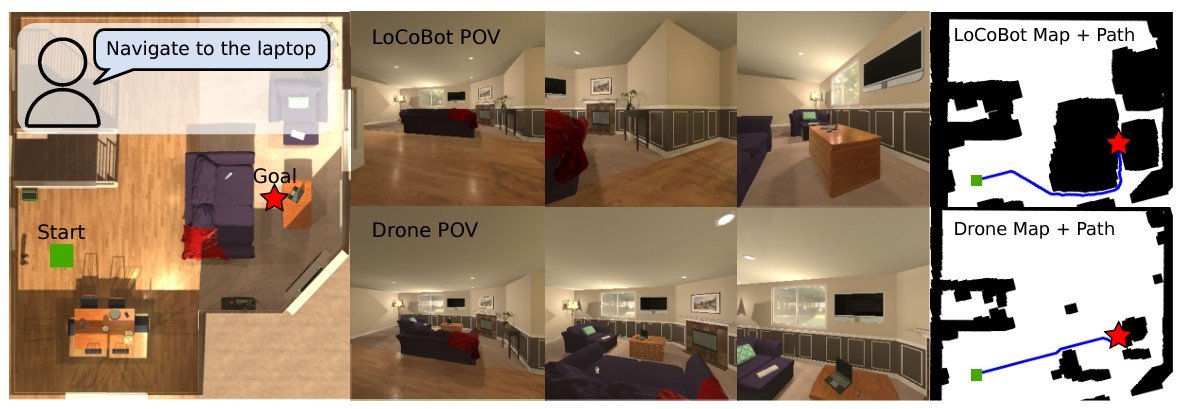
Equally, throughout testing, AVLMaps outperformed VLMaps with each commonplace AudioCLIP and wav2clip in fixing ambiguous aim navigation duties. For the experiment, robots have been made to navigate to 1 sound aim and one object aim in a sequence.
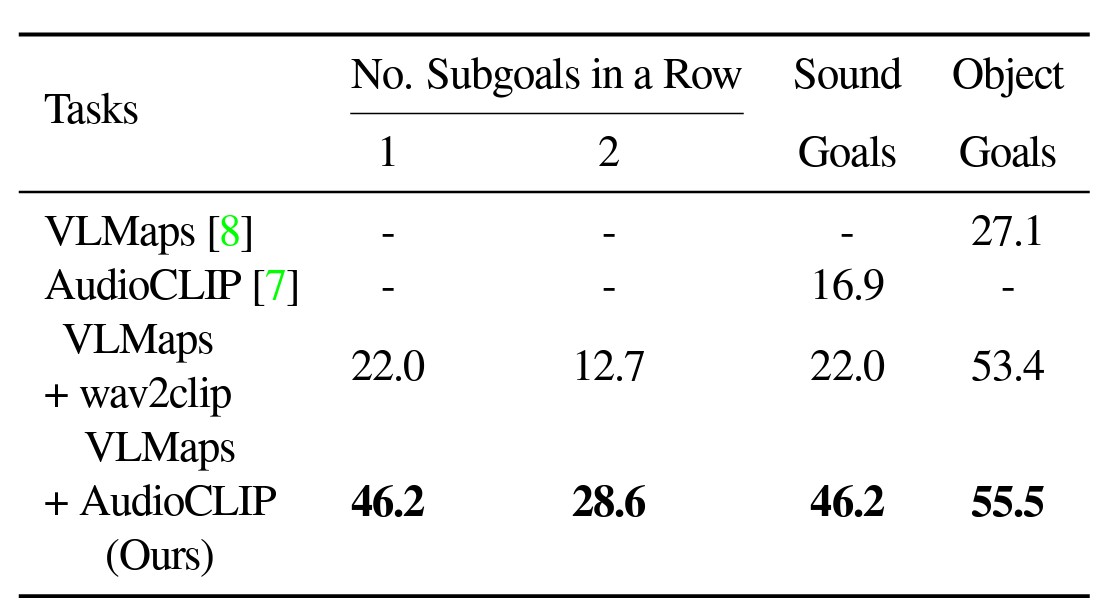
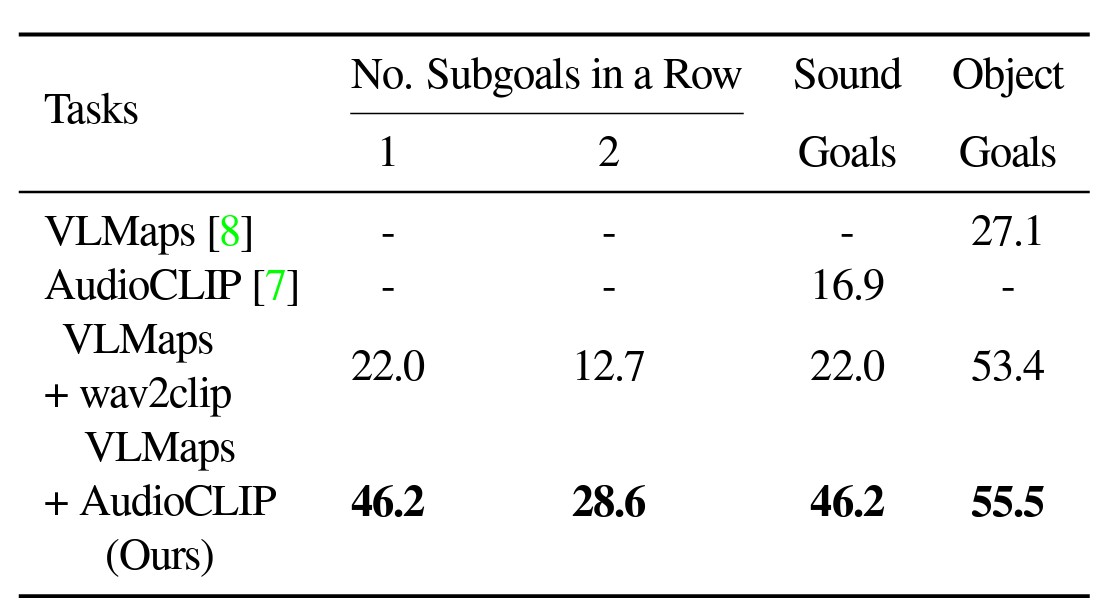
What’s Subsequent for Robotic Navigation?
Whereas fashions like VLMaps and AVLMaps present potential, there may be nonetheless an extended solution to go. To imitate the navigational capabilities of people and be helpful in additional real-life conditions, we want techniques with even larger success charges in finishing up complicated, multi-goal navigational duties.
Moreover, these experiments used primary, drone-like robotics. We’ve got but to see how these superior navigational fashions could be mixed with extra human-like techniques.
One other lively space of analysis is Occasion-based SLAM. As a substitute of relying purely on sensory info, these techniques can use occasions to disambiguate objectives or open up new navigational alternatives. As a substitute of utilizing single frames, these techniques seize adjustments in lighting and different traits to determine environmental occasions.
As these strategies evolve, we will anticipate elevated adoption in fields like autonomous automobiles, nanorobotics, agriculture, and even robotic surgical procedure.
To be taught extra concerning the world of AI and laptop imaginative and prescient, take a look at the viso.ai weblog: