Introduction
The most recent mannequin assortment from Microsoft’s Small Language Fashions (SLMs) household known as Phi-3. They surpass fashions of comparable and higher sizes on quite a lot of benchmarks in language, reasoning, coding, and math. They’re made to be extraordinarily highly effective and economical. With Phi-3 fashions out there, Azure shoppers have entry to a wider vary of wonderful fashions, offering them with extra helpful choices for creating and creating generative AI purposes. For the reason that April 2024 launch, Azure has gathered a wealth of insightful enter from customers and group members concerning areas the place the Phi-3 SLMs may use enchancment.
They’re now happy to current Phi-3.5 SLMs – Phi-3.5-mini, Phi-3.5-vision, and Phi-3.5-MoE, a Combination-of-Specialists (MoE) mannequin, as the most recent members of the Phi household. Phi-3.5-mini provides a 128K context size to enhance multilingual help. Phi-3.5-vision enhances the comprehension and reasoning of multi-frame pictures, enhancing efficiency on single-image benchmarks. Phi-3.5-MoE surpasses bigger fashions whereas sustaining the efficacy of Phi fashions with its 16 consultants, 6.6B lively parameters, low latency, multilingual help, and robust security options.
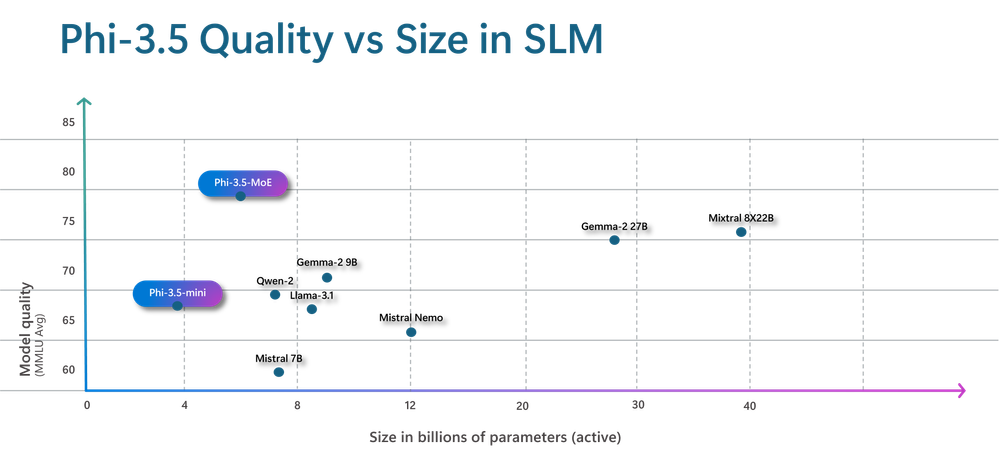
Phi-3.5-MoE: Combination-of-Specialists
Phi-3.5-MoE is the biggest and newest mannequin among the many newest Phi 3.5 SLMs releases. It includes 16 consultants, every containing 3.8B parameters. With a complete mannequin measurement of 42B parameters, it prompts 6.6B parameters utilizing two consultants. This MoE mannequin performs higher than a dense mannequin of a comparable measurement concerning high quality and efficiency. Greater than 20 languages are supported. The MoE mannequin, like its Phi-3 counterparts, makes use of a mixture of proprietary and open-source artificial instruction and choice datasets in its sturdy security post-training approach. Utilizing artificial and human-labeled datasets, our post-training process combines Direct Choice Optimisation (DPO) with Supervised High quality-Tuning (SFT). These comprise a number of security classes and datasets emphasizing harmlessness and helpfulness. Furthermore, Phi-3.5-MoE can help a context size of as much as 128K, which makes it able to dealing with quite a lot of long-context workloads.
Additionally learn: Microsoft Phi-3: From Language to Imaginative and prescient, this New AI Mannequin is Remodeling AI
Coaching Knowledge of Phi 3.5 MoE
Coaching knowledge of Phi 3.5 MoE consists of all kinds of sources, totaling 4.9 trillion tokens (together with 10% multilingual), and is a mixture of:
- Publicly out there paperwork filtered rigorously for high quality chosen high-quality instructional knowledge and code;
- Newly created artificial, “textbook-like” knowledge to show math, coding, widespread sense reasoning, common data of the world (science, day by day actions, concept of thoughts, and many others.);
- Excessive-quality chat format supervised knowledge overlaying varied subjects to mirror human preferences, akin to instruct-following, truthfulness, honesty, and helpfulness.
Azure focuses on the standard of knowledge that might doubtlessly enhance the mannequin’s reasoning skill, and it filters the publicly out there paperwork to include the proper stage of information. For instance, the results of a recreation within the Premier League on a selected day could be good coaching knowledge for frontier fashions, however it wanted to take away such info to depart extra mannequin capability for reasoning for small-size fashions. Extra particulars about knowledge could be discovered within the Phi-3 Technical Report.
Phi 3.5 MoE coaching takes 23 days and makes use of 4.9T tokens of coaching knowledge. The supported languages are Arabic, Chinese language, Czech, Danish, Dutch, English, Finnish, French, German, Hebrew, Hungarian, Italian, Japanese, Korean, Norwegian, Polish, Portuguese, Russian, Spanish, Swedish, Thai, Turkish, and Ukrainian.
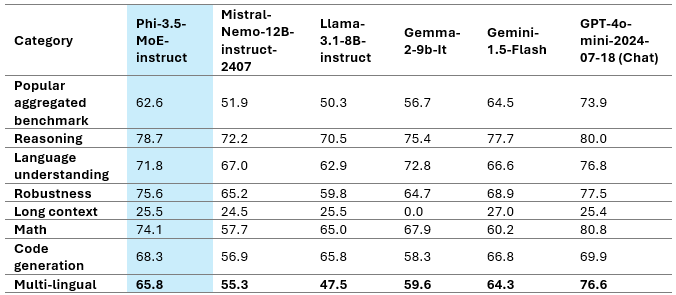
The above desk represents Phi-3.5-MoE Mannequin High quality on varied capabilities. We will see that Phi 3.5 MoE is performing higher than some bigger fashions in varied classes. Phi-3.5-MoE with solely 6.6B lively parameters achieves the same stage of language understanding and math as a lot bigger fashions. Furthermore, the mannequin outperforms larger fashions in reasoning functionality. The mannequin supplies good capability for finetuning for varied duties.
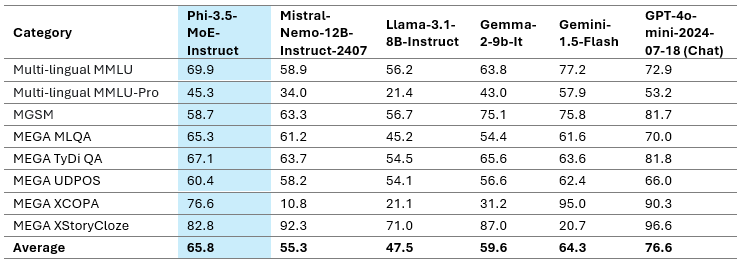
The multilingual MMLU, MEGA, and multilingual MMLU-pro datasets are used within the above desk to reveal the Phi-3.5-MoE’s multilingual capability. We discovered that the mannequin outperforms competing fashions with considerably bigger lively parameters on multilingual duties, even with solely 6.6B lively parameters.
Phi-3.5-mini
The Phi-3.5-mini mannequin underwent further pre-training utilizing multilingual artificial and high-quality filtered knowledge. Subsequent post-training procedures, akin to Direct Choice Optimization (DPO), Proximal Coverage Optimization (PPO), and Supervised High quality-Tuning (SFT), had been then carried out. These procedures used artificial, translated, and human-labeled datasets.
Coaching Knowledge of Phi 3.5 Mini
Coaching knowledge of Phi 3.5 Mini consists of all kinds of sources, totaling 3.4 trillion tokens, and is a mixture of:
- Publicly out there paperwork filtered rigorously for high quality chosen high-quality instructional knowledge and code;
- Newly created artificial, “textbook-like” knowledge to show math, coding, widespread sense reasoning, common data of the world (science, day by day actions, concept of thoughts, and many others.);
- Excessive-quality chat format supervised knowledge overlaying varied subjects to mirror human preferences, akin to instruct-following, truthfulness, honesty, and helpfulness.
Mannequin High quality
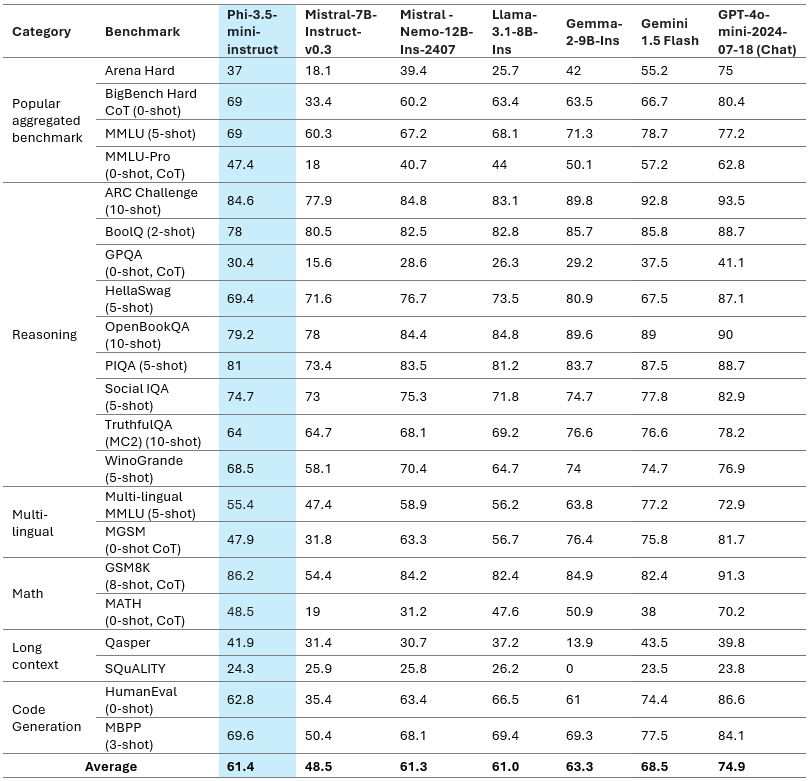
The above desk provides a fast overview of the mannequin high quality on vital benchmarks. This efficient mannequin meets, if not outperforms, different fashions with higher sizes regardless of having a compact measurement of solely 3.8B parameters.
Additionally learn: Microsoft Phi 3 Mini: The Tiny Mannequin That Runs on Your Telephone
Multi-lingual Functionality
Our latest replace to the three.8B mannequin is Phi-3.5-mini. The mannequin considerably improved multilingualism, multiturn dialog high quality, and reasoning capability by incorporating further steady pre-training and post-training knowledge.
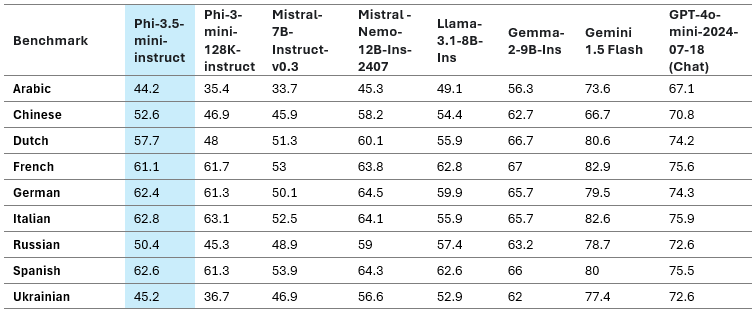
Multilingual help is a serious advance over Phi-3-mini with Phi-3.5-mini. With 25–50% efficiency enhancements, Arabic, Dutch, Finnish, Polish, Thai, and Ukrainian languages benefited essentially the most from the brand new Phi 3.5 mini. Seen in a broader context, Phi-3.5-mini demonstrates the very best efficiency of any sub-8B mannequin in a number of languages, together with English. It needs to be famous that whereas the mannequin has been optimized for greater useful resource languages and employs 32K vocabulary, it’s not suggested to make use of it for decrease useful resource languages with out further fine-tuning.
Lengthy Context

With a 128K context size help, Phi-3.5-mini is a wonderful alternative for purposes like info retrieval, lengthy document-based high quality assurance, and summarising prolonged paperwork or assembly transcripts. In comparison with the Gemma-2 household, which might solely deal with an 8K context size, Phi-3.5 performs higher. Moreover, Phi-3.5-mini has stiff competitors from significantly bigger open-weight fashions like Mistral-7B-instruct-v0.3, Llama-3.1-8B-instruct, and Mistral-Nemo-12B-instruct-2407. Phi-3.5-mini-instruct is the one mannequin on this class, with simply 3.8B parameters, 128K context size, and multi-lingual help. It’s vital to notice that Azure selected to help extra languages whereas maintaining English efficiency constant for varied duties. Because of the mannequin’s restricted functionality, English data could also be superior to different languages. Azure suggests using the mannequin within the RAG setup for duties requiring a excessive stage of multilingual understanding.
Additionally learn: Phi 3 – Small But Highly effective Fashions from Microsoft
Phi-3.5-vision with Multi-frame Enter
Coaching Knowledge of three.5 Imaginative and prescient
Azure’s coaching knowledge consists of all kinds of sources and is a mixture of:
- Publicly out there paperwork filtered rigorously for high quality chosen high-quality instructional knowledge and code;
- Chosen high-quality image-text interleave knowledge;
- Newly created artificial, “textbook-like” knowledge for the aim of instructing math, coding, widespread sense reasoning, common data of the world (science, day by day actions, concept of thoughts, and many others.), newly created picture knowledge, e.g., chart/desk/diagram/slides, newly created multi-image and video knowledge, e.g., quick video clips/pair of two comparable pictures;
- Excessive-quality chat format supervised knowledge overlaying varied subjects to mirror human preferences, akin to instruct-following, truthfulness, honesty, and helpfulness.
The information assortment course of concerned sourcing info from publicly out there paperwork and meticulously filtering out undesirable paperwork and pictures. To safeguard privateness, we rigorously filtered varied picture and textual content knowledge sources to take away or scrub any doubtlessly private knowledge from the coaching knowledge.
Phi-3.5-vision delivers state-of-the-art multi-frame picture understanding and reasoning capabilities because of important person suggestions. With a variety of purposes throughout a number of contexts, this breakthrough allows exact image comparability, multi-image summarization/storytelling, and video summarisation.
Surprisingly, Phi-3.5-vision has proven notable positive factors in efficiency throughout a number of single-image benchmarks. As an example, it elevated the MMBench efficiency from 80.5 to 81.9 and the MMMU efficiency from 40.4 to 43.0. Moreover, the usual for doc comprehension, TextVQA, elevated from 70.9 to 72.0.
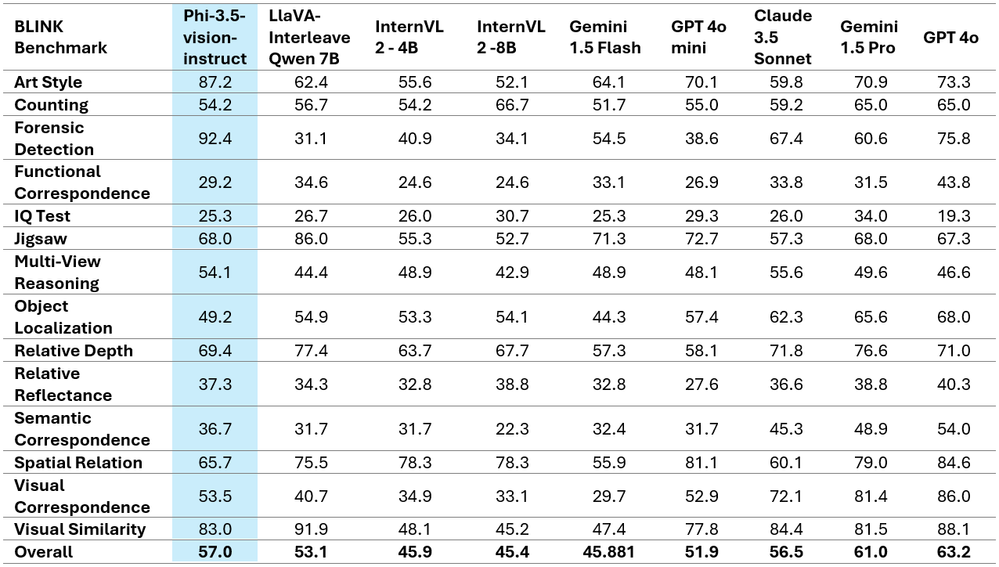
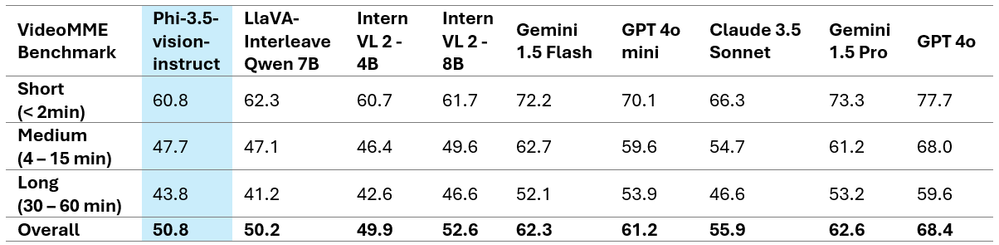
The tables above showcase the improved efficiency metrics and current the great comparative findings on two well-known multi-image/video benchmarks. It is very important word that Phi-3.5-Imaginative and prescient doesn’t help multilingual use instances. With out further fine-tuning, it’s endorsed in opposition to utilizing it for multilingual eventualities.
Attempting out Phi 3.5 Mini
Utilizing Hugging Face
We’ll use kaggle pocket book to implement Phi 3.5 Mini because it accommodates the Phi 3.5 mini mannequin higher than Google Colab. Be aware: Make certain to allow the accelerator to GPU T4x2.
1st Step: Importing needed libraries
import torch
from transformers import AutoModelForCausalLM, AutoTokenizer, pipeline
torch.random.manual_seed(0)
2nd Step: Loading the Mannequin and Tokenizer
mannequin = AutoModelForCausalLM.from_pretrained(
"microsoft/Phi-3.5-mini-instruct",
device_map="cuda",
torch_dtype="auto",
trust_remote_code=True,
)
tokenizer = AutoTokenizer.from_pretrained("microsoft/Phi-3.5-mini-instruct")
third Step: Getting ready messages
messages = [
{"role": "system", "content": "You are a helpful AI assistant."},
{"role": "user", "content": "Tell me about microsoft"},
]
“position”: “system”: Units the habits of the AI mannequin (on this case, as a “useful AI assistant”
“position”: “person”: Represents the person’s enter.
Step 4: Creating the Pipeline
pipe = pipeline(
"text-generation",
mannequin=mannequin,
tokenizer=tokenizer,
)
This creates a pipeline for textual content era utilizing the desired mannequin and tokenizer. The pipeline abstracts the complexities of tokenization, mannequin execution, and decoding, offering a simple interface for producing textual content.
Step 5: Setting Era Arguments
generation_args = {
"max_new_tokens": 500,
"return_full_text": False,
"temperature": 0.0,
"do_sample": False,
}
These arguments management how the mannequin generates textual content.
- max_new_tokens=500: The utmost variety of tokens to generate.
- return_full_text=False: Solely the generated textual content (not the enter) might be returned.
- temperature=0.0: Controls randomness within the output. A worth of 0.0 makes the mannequin deterministic, producing the most definitely output.
- do_sample=False: Disables sampling, making the mannequin at all times select essentially the most possible subsequent token.
Step 6: Producing Textual content
output = pipe(messages, **generation_args)
print(output[0]['generated_text'])
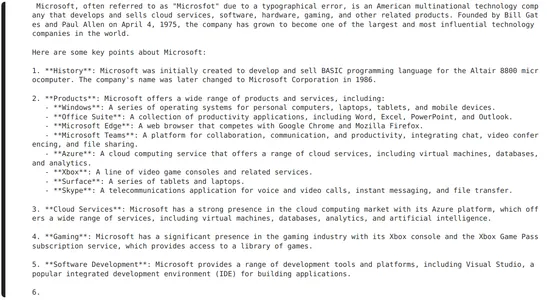
Utilizing Azure AI Studio
We will strive Phi 3.5 Mini Instruct in Azure AI Studio utilizing their Interface. There’s a part referred to as “Attempt it out” within the Azure AI Studio. Under is a snapshot of utilizing Phi 3.5 Mini.
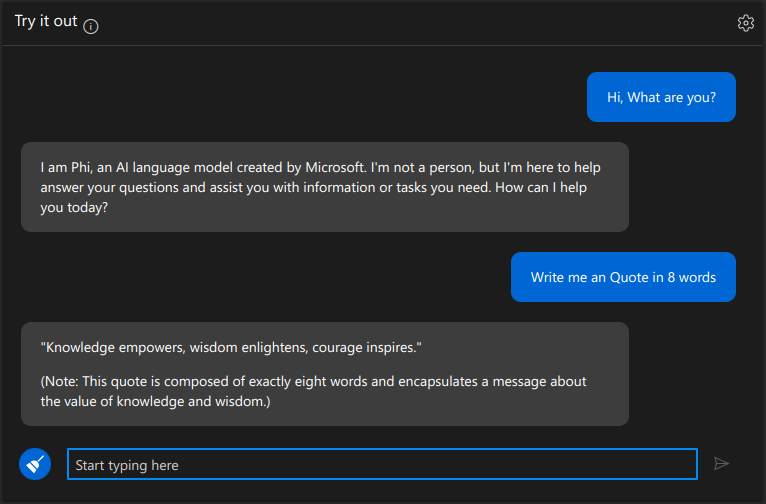
Utilizing HuggingChat from Hugging Face
Right here is the HuggingChat Hyperlink.
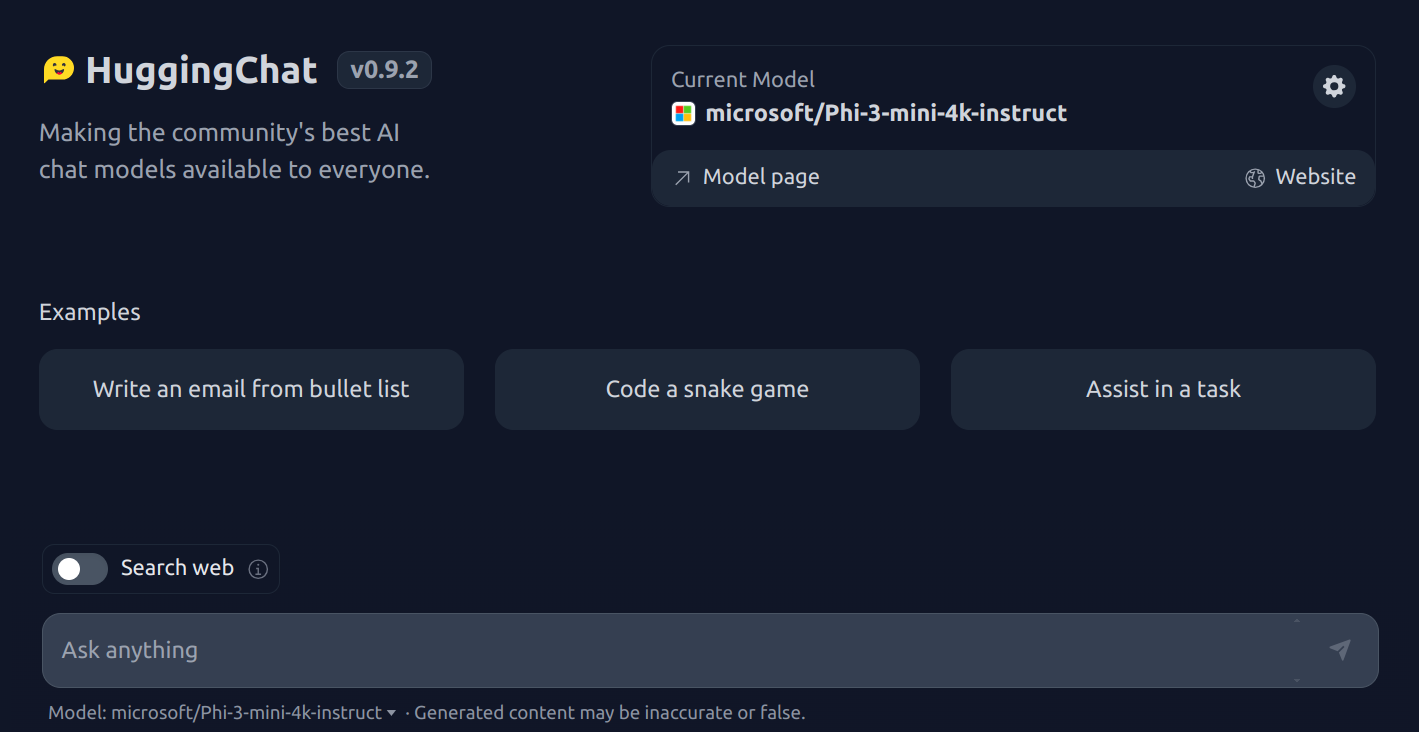
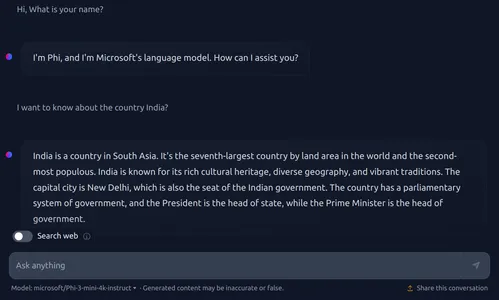
Attempting Phi 3.5 Imaginative and prescient
Utilizing Areas from Hugging Face
Since Phi 3.5 Imaginative and prescient is a GPU-intensive mannequin, we can not use the mannequin with a free tier of colab and kaggle. Therefore, I’ve used hugging face areas to strive Phi 3.5 Imaginative and prescient.
We might be utilizing the under picture.
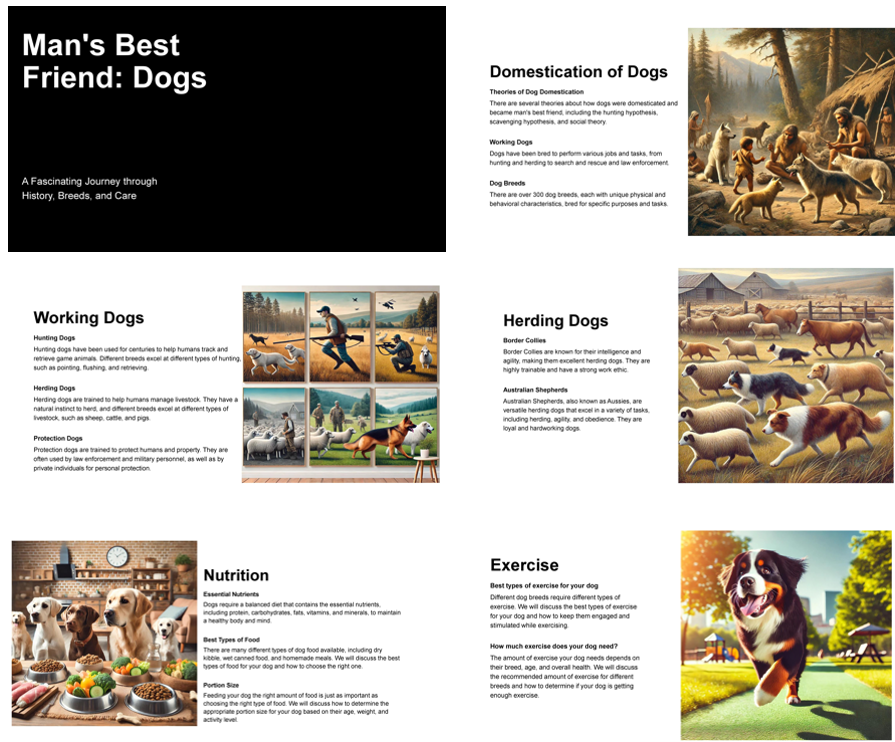
Immediate we used is “Summarize the deck of slides”
Output
The presentation is concerning the historical past and care of canines. It covers the domestication of canines, their working roles, herding talents, diet, and train. The presentation consists of pictures of canines in varied settings and actions, akin to herding sheep, working alongside people, and exercising in city environments.
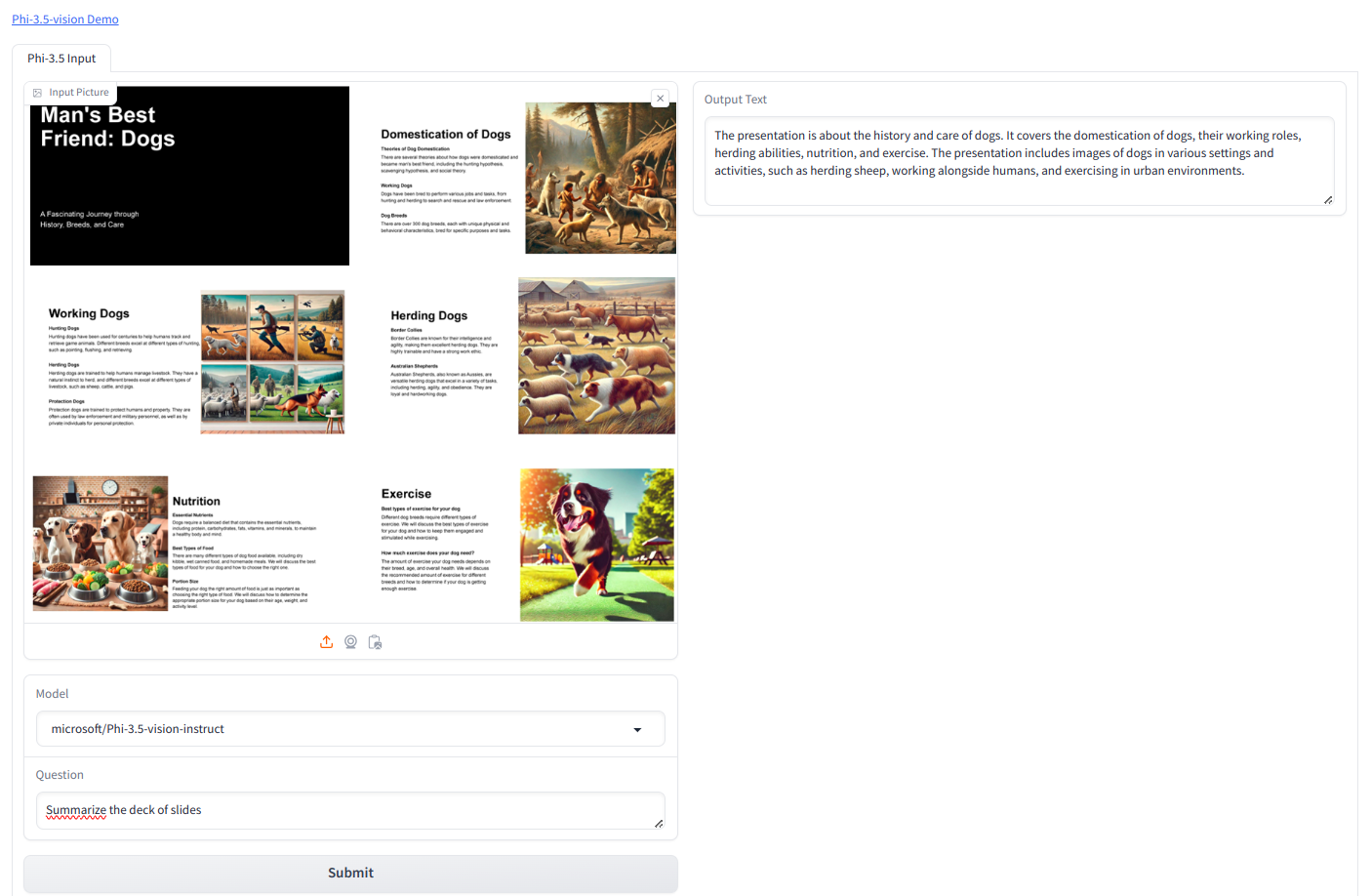
Conclusion
The Phi-3.5-mini is a singular LLM with 3.8B parameters, 128K context size, and multi-lingual help. It balances broad language help with English data density. It’s finest utilized in a Retrieval-Augmented Era setup for multilingual duties. The Phi-3.5-MoE has 16 small consultants, delivers high-quality efficiency, reduces latency, and helps 128k context size and a number of languages. It may be custom-made for varied purposes and has 6.6B lively parameters. The Phi-3.5-vision enhances single-image benchmark efficiency. The Phi-3.5 SLMs household provides cost-effective, high-capability choices for the open-source group and Azure prospects.
In case you are searching for a Generative AI course on-line, then discover in the present day – GenAI Pinnacle Program
Steadily Requested Questions
Ans. Phi-3.5 fashions are the most recent in Microsoft’s Small Language Fashions (SLMs) household, designed for prime efficiency and effectivity in language, reasoning, coding, and math duties.
Ans. Phi-3.5-MoE is a Combination-of-Specialists mannequin with 16 consultants, supporting 20+ languages, 128K context size, and designed to outperform bigger fashions in reasoning and multilingual duties.
Ans. Phi-3.5-mini is a compact mannequin with 3.8B parameters, 128K context size, and improved multilingual help. It excels in English and several other different languages.
Ans. You may strive Phi-3.5 SLMs on platforms like Hugging Face and Azure AI Studio, the place they’re out there for varied AI purposes.